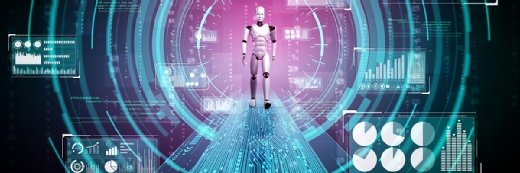
Getty Images
AI Algorithm Can Support Radiologists in Identifying Bowel Issues
A recent study described the development of a deep-learning algorithm that can help radiologists distinguish between two conditions in CT images of patients with large-bowel wall thickening.
A recent study published in JAMA Network Open described the development and use of a deep-learning algorithm that could help radiologists differentiate between colon carcinoma (CC) and acute diverticulitis (AD) within computed tomography (CT) images of patients with large-bowel wall thickening.
Radiologists often experience frequent difficulties differentiating between malignant and benign etiology when reviewing CT images showing large-bowel wall thickening; the study authors noted that artificial intelligence (AI) support systems could improve the process.
In this study, the researchers described the creation of a deep-learning algorithm to identify the differences between CC and AD within CT images. To do so, they gathered information on 585 patients who had surgery for CC or AD between July 1, 2005, and Oct. 1, 2020,
Researchers developed a three-dimensional (3D) convolutional neural network (CNN) through the delineation of 3D boxes such as the diseased bowel segment and surrounding mesentery. Following this, they led a reader study that included 10 observers of various levels of experience who were asked to classify the testing cohort with reading room conditions. They did so twice, once with algorithmic support and once without it.
The 585 patients included in the study had a mean age of 63.2 years, and 341 were men. Researchers considered factors such as sensitivity, specificity, positive predictive value (PPV), and negative predictive value (NPV) for readers and reader groups as they evaluated diagnostic performance. To ensure valuable data collection, they calculated these measures with and without AI support.
They found that the 3D CNN achieved a sensitivity of 83.3 percent and a specificity of 86.6 percent for the test set. However, the mean researcher reported a sensitivity of 77.6 percent and a specificity of 81.6 percent.
They also found that the combined group of readers improved with AI support as sensitivity rose from 77.6 percent to 85.6 percent. Along with this, specificity rose from 81.6 percent to 91.3 percent. Additionally, AI support led to reductions in false-negative and false-positive findings.
Based on these findings, researchers concluded that a deep-learning algorithm could successfully differentiate between CC and AD in CT images, providing researchers with an effective support tool.
But, the study has several limitations, including a potential lack of generalizability and the inclusion of only common malignant and harmless entities for bowel wall thickening. In future studies, the model should be adapted for malignant and benign entities in general, the researchers stated.
The use of deep-learning strategies to improve the accuracy and efficiency of medical practices is growing.
In January, for example, researchers validated a deep-learning model that aimed to predict lung cancer risk using chest radiographs and EMR information.
The tool was designed to identify high-risk smokers for lung cancer screening CT scans using readily available EMR data, including age, sex, current cigarette smoking status, and chest radiograph image.
After collecting the data and developing the tool, researchers tested it and concluded that the model could accurately identify patients at high risk for lung cancer.