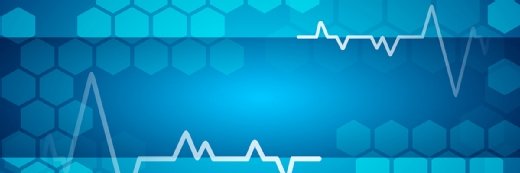
Ace2020/istock via Getty Images
Predictive Analytics Tool Identifies Readmission Risk, Reduces Costs
In a study, Corewell Health researchers described how predictive analytics helped the organization prevent 200 patient readmissions and drive $5 million in cost savings.
Corewell Health care coordinators shared that a recent initiative, which uses predictive analytics to forecast risk and reduce readmissions, has kept 200 patients from being readmitted and resulted in a $5 million cost savings.
These results, initially reported in a study published in NEJM Catalyst in December, stemmed from an initiative that began in January 2021 when the care coordination team at Spectrum Health, now part of Corewell Health, started to predict readmission risk. Leveraging artificial intelligence and predictive analytics, the team looked at which patients faced a more difficult recovery after their hospitalization and used that information to create a plan to address barriers to recovery from the first day of discharge to the end of the first month.
“By working in advance of recovery barriers and focusing on whole-person needs, real rates of readmission can be reduced, even for people at high risk for return to acute care,” said study co-author Alejandro Quiroga Chand, MD, senior vice president, chief medical officer ambulatory care and population health, Corewell Health West, in the press release.
According to the press release, patients at the greatest risk of readmission received more targeted transition support and interventions that aimed to address three aspects of recovery: clinical challenges, behavioral health, and social determinants of health (SDOH).
Once these patients were identified, interdisciplinary teams worked on mapping interventions over the month of transition support to meet each patient’s personal needs. Interventions relied on task-oriented follow-up and proactive outreach to achieve these goals, incorporating questions and support related to behavioral health, transportation, diet, and other factors.
These interventions had a significant impact on care coordination and delivery.
“In addition to the benefit in care delivery for individual patients, this initiative has had a beneficial impact in terms of performance on targeted U.S. Centers for Medicare & Medicaid Services readmissions and value-based contract arrangements,” said study co-author James Moses, MD, chief quality, safety and experience officer, Corewell Health, in the press release.
Based on the results of this 20-month initiative, the researchers concluded that these efforts are both sustainable and scalable. The program will be expanded this year from 15 offices to 45 primary care sites that serve patients enrolled with Priority Health, the press release states.
Recently, predictive analytics has seen significant interest from health systems looking to tackle hospital readmissions.
In November, researchers revealed that they had developed a suite of predictive analytics tools to reliably identify all-cause 30-day pediatric readmission risk before hospital discharge.
To model all-cause 30-day readmission, the research team leveraged readily available EHR data and used ongoing length of stay (LOS), use of specific therapies, and past utilization as predictive factors. These were used to generate three models for prediction: a recent admission model (RAM), which included children 6 months or older with one or more prior hospitalizations within the last six months; a new admission model (NAM), which included children 6 months or older with no prior hospitalizations in the last six months; and a young infant model (YIM), which included children younger than 6 months.
The models were designed to work together as a suite of tools to predict pediatric readmission risk. They achieved acceptable to excellent performance across cohorts. The researchers concluded that while further research is needed, similar risk prediction models may improve discharge preparedness and prevent high-risk readmissions in the future.