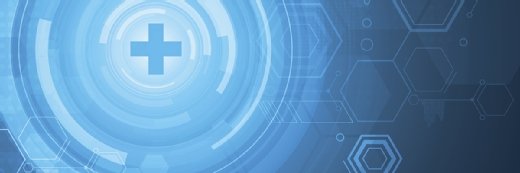
traffic_analyzer/DigitalVision V
Machine-Learning Model Provides Predictions on Physician Turnover
A recent study described a machine-learning model that could determine physician turnover with 97 percent accuracy and helped identify factors linked to departure risk.
A recent study by Yale University researchers detailed the creation of a machine-learning model that can assist researchers in determining physician turnover, enabling healthcare organizations to intervene and increase retention.
Physician turnover is challenging for patients and healthcare facilities alike. Though there are ways to track job satisfaction, such as surveys, the burden is on physicians to respond, and as a result, response rates can be low, the press release noted.
In this study, which was published in PLOS ONE, researchers aimed to use EHR data to evaluate physician behavioral patterns to determine turnover trends. The data that researchers used for the study included three years’ worth of deidentified EHR and physician data from a New England healthcare system. Their goal was to figure out if they could use a three-month period of information to determine the chances of a physician leaving a position within the coming six months.
“We wanted something that would be useful on a personalized level,” said Andrew Loza, MD, PhD, a lecturer and clinical informatics fellow at Yale School of Medicine and co-senior author of the study, in a press release. “So if someone were to use this approach, they could see the likelihood of departure for a position as well as the variables contributing most to the estimate in that moment, and intervene where possible.”
Researchers collected data from 319 physicians representing 26 medical specialties over 34 months. They focused on various measures, including time spent using EHRs, clinical productivity measures, and physician characteristics.
The model testing led researchers to conclude that it was 97 percent accurate in predicting physician departure. This trial also reported that the model achieved a sensitivity of 64 percent and a specificity of 79 percent.
In addition to the reliability associated with the model, it also displayed the ability to identify variables that may contribute to turnover risk, how they related to one another, and how they changed when a physician went from low risk of departure to high risk.
Researchers found that length of physician employment, age, the complexity associated with their cases, and the extent to which their services were demanded were all factors associated with departure risk.
“There have been efforts to make machine learning models not black boxes wherein you get a prediction but it’s not clear how the model came to it,” said Loza in a press release. “Understanding why the model produced the prediction it did is particularly useful in this case as those details are going to identify issues that may be leading to physician departure.”
The use of machine learning for prediction is becoming a common practice in clinical care.
For example, a study from December 2022 described how the addition of preoperative and intraoperative hemodynamic monitoring data to a machine learning-based prediction model led to accurate predictions of the need for massive transfusion during surgery.
Another study from November 2022 described how machine learning could determine the timeline for the advancement of Alzheimer’s disease among those considered cognitively normal or those who experience mild cognitive impairment. To do this, the research team used machine learning to analyze biomedical data from patient care journeys.
As a result, they gained several insights into accurately predicting cognitive decline. They also found that predicting the decline into dementia is easier for those with mild cognitive impairment than for those who are cognitively normal.