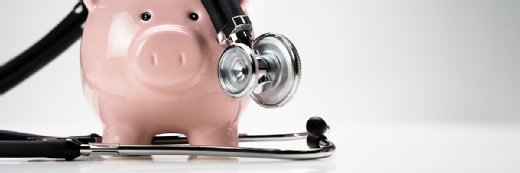
AndreyPopov/istock via Getty Ima
Machine-Learning Intervention Leads to Safer Cardiac Catheterization
New research shows that a machine-learning intervention resulted in a 46 percent lower likelihood of acute kidney injury from cardiac catheterization.
As reported in the Clinical Journal of the American Society of Nephrology, a randomized trial conducted by Vanderbilt University Medical Center (VUMC), the Dartmouth Geisel School of Medicine, and the Larner College of Medicine at the University of Vermont found that a machine-learning intervention provided a higher level of safety during cardiac catheterization, lowering the chances of acute kidney injury (AKI).
According to the study, over 1 million cardiac catheterizations occur in the US annually. Ranging from diagnostic to therapeutic purposes, this procedure consists of threading a thin tube through the circulatory system into a chamber or vessel of the heart.
However, there are downsides to the procedure, including the possibility of AKI, which as many as 14 percent of patients experience.
In an attempt to lower AKI rates during these procedures, researchers from three institutions compared four improvement strategies across several Veterans Health Administration medical centers. These four strategies included a modicum of technical coaching alone, a more thorough virtual learning program alone, and the two methods separately wedded with an electronic dashboard with month-to-month observed-to-expected AKI statistics.
The electronic dashboard feature was powered by an AKI prediction algorithm that used machine learning and information from EHRs.
“While some interventions, such as hydration and limiting contrast, are well known to reduce AKI risk, it can be challenging for all facilities to implement identical protocols,” said Michael Matheny, MD, a leader of the study, internal medicine specialist and observational data scientist, and professor of biomedical informatics at VUMC, in a press release. “This implementation trial facilitated each site team to make their own decisions based on quality improvement coaching and availability of case series, outcomes, and awareness of local case mix. It was really exciting to see different clinical foci and different process changes across sites show improvements in overall AKI event rates through the use of the various interventions.”
The total number of cardiac catheterization patients included in the study was 4,517, of whom 510 experienced AKI after the procedure.
Researchers found that among the medical centers that used technical coaching or virtual learning exclusively, the AKI rate was 13 percent. For those that used the outcomes dashboard that was wedded to light technical coaching, the rate was 11 percent. When the dashboard was wedded with virtual learning, the rate was 8 percent.
According to the study, the 5 percent difference between 13 and 8 percent is equivalent to a 38 percent drop in AKI. Also, in data adjusted for patient severity, this is estimated to equal a 46 percent reduction in risk for AKI.
Machine learning is increasingly being used in clinical predictive analytics.
A similar intervention in January consisted of Johns Hopkins University researchers creating two machine-learning algorithms to detect early warning signs of delirium and predict the patients at risk for developing delirium during an intensive care unit stay.
The first static model used a single snapshot of patient data and considered age, illness severity, and other factors to predict risk.
The second model was dynamic and monitored patient data over the course of hours and days following admission while using information such as pulse and temperature to determine delirium risk.
They found that the static model could accurately predict which patients would experience delirium 78.5 percent of the time. The dynamic model achieved a higher performance score and could predict delirium-prone patients up to 90 percent of the time.