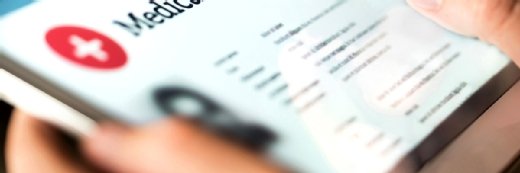
terovesalainen - stock.adobe.com
Researchers Identify Social Predictors of Premature Death Risk for Seniors
Leveraging machine-learning approaches, new research identified eight social factors that predict premature death for older adults, including feeling isolated and not working for pay.
Researchers at Massachusetts General Hospital (MGH) and the University of California, San Francisco have identified eight social characteristics that can help to predict an older adult’s risk of premature death using machine-learning (ML) methods.
According to the study discussing these findings, published in the Proceedings of the National Academy of Sciences (PNAS) last week, social factors are known to impact an individual’s future health, but practical approaches to summarize the prognostic impacts of such factors are limited.
To combat this, the researchers aimed to take a comprehensive look at older adults’ social attributes, identify those that had the most significant impact on longevity, and use them to predict premature death risk.
The research team analyzed data from 8,250 adults ages 65 and older to identify potential social predictors using an ML method known as LASSO. The analysis generated 183 possible social predictors, and, gaining insights from the 22 percent of the cohort that died within four years of the study’s baseline interview, revealed eight factors deemed most significant for predicting premature death: poor neighborhood cleanliness, low perceived control over financial situation, meeting with children less than yearly, not working for pay, not active with children, not volunteering, feeling isolated, and being treated with less courtesy or respect.
“We often overemphasize the importance of medical conditions when thinking about longevity. This research demonstrates that our social lives are as important as medical conditions,” said lead author of the study Sachin J. Shah, MD, a physician-scientist at MGH and Harvard Medical School, in the press release.
These factors were then used to develop a 10-question survey called the Social Frailty Index, which leverages gender, age, and social characteristics to predict longevity and other outcomes relevant when looking at older adult populations, such as living independently.
"Failing to account for social risks can further social inequities," said senior study author Kenneth E. Covinsky, MD, a clinician-researcher in the Division of Geriatrics at UC San Francisco, in the press release. "Medicare penalizes hospitals for excess readmissions, as determined by a risk adjustment model. By ignoring social factors, Medicare penalizes those hospitals serving socially marginalized communities."
The researchers indicated that they are working to validate their findings and incorporate the Social Frailty Index into additional medical research. But the survey is currently available online to supplement other commonly used prediction tools, which may help older adults engage in advanced care planning and consider various medical interventions.
They also cautioned that the tool should not be used to limit the care of higher-than-average-risk older adults because a patient is “socially frail” but instead to identify at-risk individuals who could benefit from measures to address health-related social issues.
The research is the most recent addition to a group of projects leveraging artificial intelligence (AI) and analytics to tackle challenges related to aging and health disparities.
Last month, the Massachusetts AI and Technology Center for Connected Care in Aging and Alzheimer’s Disease (MassAITC) at the University of Massachusetts (UMass) Amherst announced $1.7 million in funding for seven projects to pioneer approaches for in-home care using AI and other advanced technologies.
The pilot projects aim to support the goal of aging at home through an interdisciplinary research approach that leverages the perspectives of patients, caregivers, clinicians, behavioral scientists, and others. The projects will focus on enhancing efforts in wearable and contactless sensing, AI, and ML to address challenges related to aging and managing Alzheimer's disease and related dementias (AD/ADRD).