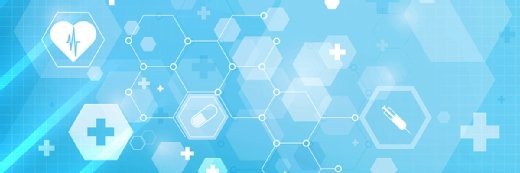
Rifqyhsn Design/istock via Getty
ChatGPT Passes US Medical Licensing Exam Without Clinician Input
ChatGPT achieved 60 percent accuracy on the US Medical Licensing Exam, indicating its potential in advancing artificial intelligence-assisted medical education.
Researchers from Massachusetts General Hospital (MGH) and AnsibleHealth, a technology-enabled medical practice providing care to medically complex chronic respiratory disease patients, found in a recent study that the artificial intelligence (AI) chatbot ChatGPT can pass the United States Medical Licensing Exam (USMLE) — findings that may highlight the tool’s potential use cases in medical education.
According to an MGH research spotlight describing the findings, ChatGPT is an advanced AI chatbot developed by OpenAI and released to the public late last year. The tool is a generative large-learning model (LLM), a type of machine learning designed to perform natural language processing (NLP) tasks by analyzing large amounts of text and other language data to find patterns and relationships within, the study explains. Following training, the model can generate new text based on the text it was trained on.
The text generated by ChatGPT can mimic that written by a human and be used to answer questions, translate text, summarize information, and generate stories by using the patterns identified in the training data to anticipate the next words in a phrase or sentence. However, ChatGPT, unlike other AI chatbots, cannot search the web to inform its predictions, relying entirely on its training data.
Recent hype and concerns around the use of ChatGPT in healthcare, such as digital mental health service Koko’s reported use of the tool in an experiment to help develop responses to users, have raised questions about its use cases.
To evaluate one aspect of ChatGPT’s potential utility, the researchers evaluated its performance on the USMLE, which consists of three standardized tests that medical students must pass to obtain a medical license.
To do this, the research team obtained publicly available test questions from the June 2022 sample exam released on the official USMLE website. Questions were then screened, and any question requiring visual assessment was removed.
From there, the questions were formatted in three ways: open-ended prompting, such as ‘What would be the patient’s diagnosis based on the information provided?’; multiple choice single answer without forced justification, such as ‘The patient's condition is mostly caused by which of the following pathogens?’; or multiple choice single answer with forced justification, such as ‘Which of the following is the most likely reason for the patient’s nocturnal symptoms? Explain your rationale for each choice.’
Each question was then put into the model separately to reduce the tool’s memory retention bias.
During testing, the researchers found that the model performed at or near the passing threshold of 60 percent accuracy without specialized input from clinician trainers. They stated that this is the first time AI has done so.
The researchers also discovered upon evaluating the reasoning behind the tool’s responses that ChatGPT displayed understandable reasoning and valid clinical insights, which led to increased confidence in trust and explainability.
The research team suggests that these findings highlight how ChatGPT and other LLMs may potentially assist human learners in medical education and be integrated into clinical settings, like AnsibleHealth’s ongoing efforts to translate technical medical reports into more easily understandable language for patients using ChatGPT.
Other research has also sought to leverage NLP to improve patient outcomes.
In September, the University of California, Irvine (UCI) and software company Melax Tech launched a partnership to enable UCI researchers to analyze EHR data using NLP to improve patient safety and outcomes.
Under the partnership, UCI will incorporate Melax Tech’s NLP products into the UCI Health Data Science Platform, allowing researchers to analyze free-text clinical data. The researchers will also use Melax Tech’s LANN tool to annotate clinical notes and train NLP models, as well as the company’s CLAMP tool to develop and customize models with various rules and features for clinical information extraction. Further, the collaboration will help validate any resulting models using large-scale EHR datasets and provide additional research opportunities.