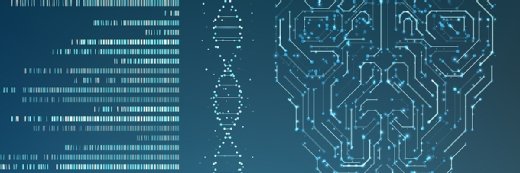
Nobi_Prizue/istock via Getty Ima
University of Utah AI, Machine Learning Projects Receive Research Funding
A set of grants supported by the New One Utah Data Science Hub will support seven University of Utah artificial intelligence and machine learning projects.
Seven research projects from the University of Utah received grants from One Utah Data Science Hub to advance efforts surrounding the use of artificial intelligence (AI) and machine learning (ML) in basic and health science.
According to the press release, data science is an umbrella term that includes data management, analytics, and mining, as well as machine learning, visualization, and other related subjects.
One Utah Data Science Hub is an effort that aims to improve research and training surrounding data science. The hub enables researchers to conduct cross-campus and interdisciplinary studies that use data science through the launch of two new initiatives: the Data Science and Ethics of Technology (DATASET) Initiative and the Data Exploration and Learning for Precision Health Intelligence (DELPHI) Initiative.
The seven projects from the university to receive seed funding from the Hub include research on the use of AI and ML to solve issues related to health sciences. Each of these projects will receive up to $50,000 for one year.
"We are thrilled about these diverse data science research projects because of their clear potential for innovation and the exciting new collaborative research teams that have been formed," said Aaron Quinlan, PhD, co-director of the DELPHI Initiative and professor of human genetics, in a press release.
The first project, Individualization of Fetal Growth Assessment using Maternal Genetics and Explainable AI, aims to create an AI-based neonatal morbidity risk calculator to track fetal growth. With this tool, researchers plan to establish a new standard for informed decision-making in obstetric scenarios.
The second project, A Novel Approach to Visualizing Pollution Exposure Patterns in Pregnant Women, will use ML to create self-assembling maps to assist healthcare professionals in tracking air pollutants and their effect on pregnancy.
Another project, known as Use of Modularity Optimization to Define and Evaluate Regional Networks for Emergency General Surgery Care, aims to use an innovative network science method to identify emergency general surgery (EGS) care trends. According to researchers, EGS results in over 3 million hospital admissions yearly, accounting for half of all surgery-related deaths.
A project known as CURATE Sepsis: CURating A DaTabase from the Electronic Health Records of Patients At-Risk for Sepsis will gather the EHR data of about 100,000 potential sepsis patients admitted to Intermountain Healthcare, creating a wide-ranging dataset for future analyses.
In addition, a project called Machine Learning-Based Heterogeneous Treatment Effects Estimation of 2nd-line Medication Options for Type 2 Diabetes Patients Using Veteran Affairs Electronic Health Record Data will use EHR data from Veteran Affairs health facilities to develop new ML-based approaches to determine treatment methods for mitigating hypoglycemia risks among type 2 diabetes patients.
Two other projects — Optimizing Across the Rashomon Set and Predicting Perturbation Phenotypes in the Vertebrate Brain — will focus on understanding the Rashomon Effect's impact on research and the relationships between brain building and brain function. The Rashomon Effect occurs when several ML models provide similar results but with varying explanations.
AI is increasingly being applied to clinical care. In April 2022, Yale School of Medicine researchers created an AI model that uses electrocardiogram (ECG) images to diagnose heart rhythm and conduction disorders.
Based on data from over 2 million ECGs, the AI model displayed the ability to diagnose one in six patients with a heart rhythm disorder. Researchers also noted that the AI approach could monitor several clinical diagnoses simultaneously.