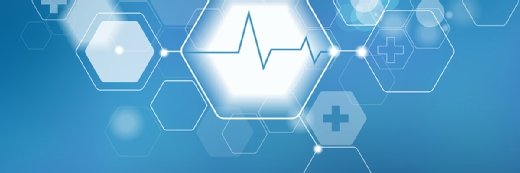
AlfaOlga/istock via Getty Images
AI, Remote Data Capture Partnership to Advance Type 2 Diabetes Research
UW Medicine is collaborating with Delve Health to use artificial intelligence to identify factors that promote and preserve the well-being of patients with Type 2 diabetes.
UW Medicine and Delve Health have announced a collaboration aimed at using artificial intelligence (AI), machine learning (ML), and remote data capture to bolster endemic type 2 diabetes research.
The partnership will leverage Delve Health's digital healthcare platform for decentralized clinical trial research and remote patient monitoring, known as Clinical StudyPal, to monitor, track, analyze, and report patient data, such as heart rate, activity levels, and blood oxygen saturation (SpO2 ) via a smartwatch. These data will then be reported to the clinical study team at UW Medicine, according to the press release.
The trial is set to recruit a cross-sectional dataset of over 4,000 people from across the US with four stages of diabetes severity: no diabetes, lifestyle controlled, oral medication controlled, and insulin dependent.
The press release also notes that the cohort will be intentionally balanced across self-reported White, Black, Asian-American, and Hispanic race/ethnicity groups by recruiting an equal number of individuals in each group rather than targeting the demographic makeup of the US population. The same approach will be applied across each of the four diabetic severity categories.
Part of the goal of this research is to identify factors that promote and preserve well-being, an approach to health known as salutogenesis.
"Our NIH-funded Bridge2AI project called Artificial Intelligence Ready Equitable Atlas for Diabetes Insight [AI-READI] will collect and release a flagship medical dataset for salutogenesis that will hopefully accelerate machine learning applications and generate novel hypotheses about Type 2 Diabetes Mellitus," said Aaron Lee, MD, an associate professor in ophthalmology at the University of Washington School of Medicine, in the press release. "As part of this dataset, we are collecting wearable fitness tracking data along with continuous glucose monitoring to build a biophysical profile of each participant. The smart watches used in this study will hopefully provide both activity monitoring and heart rate measurements that will be critical for achieving this aim."
Other recent research has also looked to AI to help improve diabetes care.
In November, researchers at the University of Houston shared that they had developed an AI-based clinical decision support (CDS) tool, known as Primary Care Forecast, that uses deep learning (DL) to predict which patients are more likely to experience diabetes complications.
The tool will be used to help enhance the utility of the Diabetes Complication Severity Index (DCSI) Progression Tool, which uses patient health history alongside social and environmental factors — such as employment status, living arrangement, education level, and food security — which can impact disease progression to evaluate the patient risk for diabetes complications.
The DCSI is a risk stratification tool that has previously been used to quantify patient complications at a single point in time, but it cannot predict which patients are at the highest risk for rising DCSI scores. Primary Care Forecast is designed to help fill this gap by informing clinicians why patients are at risk and suggesting actions to reduce that risk.