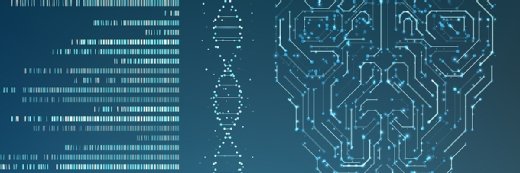
Nobi_Prizue/istock via Getty Ima
AI Model Provides Insights into Long-Term Traumatic Brain Injury Risk
A new AI model may signal a ‘paradigm shift’ in traumatic brain injury research by more accurately modeling the tissue deformations that lead to brain damage.
Stanford University researchers are leveraging artificial intelligence (AI) to help identify which computational models perform best at modeling mechanical stress on the brain, which may help drive insights into why some traumatic brain injuries (TBIs) lead to long-term brain damage while others do not.
The press release states that the ability to model the mechanical forces causing the compression, stretching, twisting, and other deformations of brain tissue that lead to brain damage is critical to understanding TBI. This modeling could help researchers understand why some TBIs lead to lasting brain damage and some don’t.
“The problem in brain modeling to date is that the brain is not a homogeneous tissue – it’s not the same in every part of the brain. Yet, trauma is often pervasive,” said Ellen Kuhl, PhD, professor of mechanical engineering, director of Stanford’s Living Matter Lab, and senior author of the study, in the press release. “The brain is also ultrasoft, much like Jell-O, which makes both testing and modeling physical effects on the brain very challenging.”
The model, known as Constitutive Artificial Neural Networks, takes a “model-of-models” approach to identify which material model out of over 4,000 best explains the behavior of the brain. Previously, this process was complicated because of the sheer number of possibilities, variations in user experience and personal preference, and the fact that one model may work for a certain type of stress on the brain but not another, the press release explains.
To address this, the tool removes some of these challenges and automates the process.
“We take user selection out of the equation by allowing machine learning to examine the data and decide which model works best,” added Sarah St. Pierre, a doctoral scholar in Kuhl’s lab and a co-author of the paper, in the press release. “Automating this process lowers barriers to model the brain.”
Once the tool selects the best model to represent a particular type of stress on the brain, that model can then be related to other models to help researchers gain new insights into TBI.
“We provide the network all existing constitutive models developed over the last century. And the AI does a mix-and-match to find the best option. This is impossible to do by hand,” explained Kevin Linka, a postdoctoral fellow in mechanical engineering working in the Living Matter Lab, who developed the tool, in the press release. “Now, we’ve effectively discovered a new model that makes us more confident in studying the mechanical stress in the brain.”
For example, using the approach, the research team has identified physically meaningful parameters, such as stiffness, in different areas of the brain. Stiffness, or shear modulus, highlights how the force from a hit to the head relates to the resulting deformation of the brain tissue.
The finding that various parts of the brain have different stiffness – the cortex, basal ganglia, corona radiata, and corpus callosum have shear stiffness of 1.82, 0.88, 0.94, and 0.54 kilopascals, respectively – can help researchers more accurately understand where brain trauma originates, which may lead to improvements in protective equipment and medical treatments.
“What’s really most exciting about this research,” Kuhl said, “is that Constitutive Artificial Neural Networks could induce a paradigm shift in soft tissue modeling, from user-defined model selection to automated model discovery. This could forever change how we simulate materials and structures.”