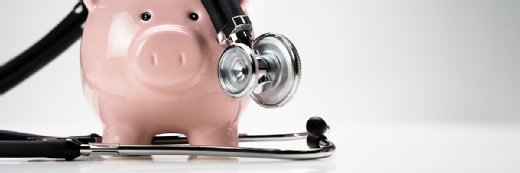
AndreyPopov/istock via Getty Ima
NLP Tools Predict Cancer Survival Using Routinely Collected Data
Natural language processing tools accurately predicted cancer survival at six, 36, and 60 months using patients’ initial oncologist consultation documents.
Researchers have found that natural language processing (NLP) tools can predict the survival of patients with cancer using their initial oncologist consultation document without additional data, according to a study published last month in JAMA Network Open.
Cancer is a significant cause of morbidity and mortality, creating significant healthcare burdens worldwide. Improving care and patient outcomes is the subject of an extensive body of medical research, but predicting outcomes for cancer patients and advancing these goals can be challenging.
The researchers explained that predicting survival rates for cancer patients relies on various factors, such as cancer site and histology, and calculations are made retrospectively. Human error can also come into play, as oncologists may struggle to account for additional considerations, like age, when predicting cancer survival for an individual patient.
Machine learning (ML) has been applied to predict various disease states and outcomes, including cancer, but the study authors noted that there is a gap in the literature regarding the application of NLP methods in cancer research generally and, more specifically, in cancer survival prediction.
To address this, the research team sought to develop and assess neural NLP models to predict survival among patients with general cancer using their initial oncologist consultation documents, which contain routinely collected information gathered during that first appointment. They also stated that in doing so, they aimed to create a tool that could predict the outcome of more than one type of cancer and not rely on data that is often limited or unavailable, which are challenges faced when using other models.
They began by gathering retrospective prognostic data from 47,625 patients who started cancer care and had oncologist consultation documents generated within 180 days of diagnosis at six hospitals in British Columbia, Canada, from April 1, 2011, to Dec. 31, 2016.
Mortality data for these patients were updated until April 6, 2022, and patients with more than one type of cancer were excluded from the study. Cancer survival was calculated as the number of months between the selected document’s creation and either the patient’s recorded death date or April 6, 2022, when mortality data were last extracted.
Consultation documents were then preprocessed to be used by the models, and binary labels were created to denote whether the patient survived at six, 36, or 60 months or did not survive. This data processing was necessary because language models are used to assign probabilities to the order of words, helping them predict a particular sequence of words or determine if one sequence is more likely than another, the researchers explained.
This task can be applied to predicting binary survival outcomes. To assess whether one type of language model would perform significantly better than another, the researchers compared four of them: a non-neural NLP, a convolutional neural network (CNN), a long short-term memory (LSTM) model, and a bidirectional encoder representations from transformers (BERT) model.
Overall, 87 percent of the study cohort survived six months, 65.4 percent survived 36 months, and 58.5 percent survived 60 months after their initial consultation.
Model performance was evaluated in terms of prediction accuracy and quantified using area under the curve. All models achieved high performance, but differences were noted across survival intervals. The models achieved an average AUC of 0.928 for predicting six-month survival, 0.918 for 36-month survival, and 0.918 for 60-month survival.
These performances were comparable or superior to those of other models, the researchers indicated, suggesting that it may be possible to develop a clinically useful tool for survival prediction that is not limited to one type of cancer and can utilize data that are readily accessible.