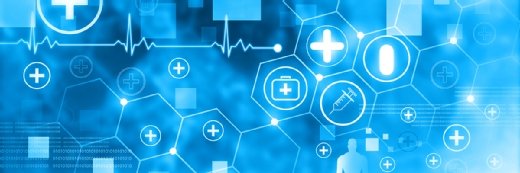
Rasi Bhadramani/istock via Getty
Artificial Intelligence System Predicts Postoperative Complications
A recent study found that an artificial intelligence system had similar predictive abilities to surgeons and could be effectively integrated into clinical workflows.
Recent research published in JAMA Network Open found that an artificial intelligence (AI) system known as MySurgeryRisk used EHR data and input features to predict postoperative complications successfully.
Predicting postoperative complications is extremely valuable in informing clinical decision-making during surgical procedures. These predictions can assist providers in determining the efficacy of specific procedures, strategies, and resource use post-operation.
However, researchers posited that AI-based predictive analytics could successfully use EHR data to predict postoperative complications and that the automated outputs could be delivered directly to surgeons' mobile devices, integrating the predictions within surgeon workflows.
Thus, this study focused on determining whether an AI system known as MySurgeryRisk could perform successfully in the development phase and if this remained stable in the validation phase. Researchers also aimed to determine whether the system could provide prediction outputs to surgeons' mobile devices.
Researchers noted their use of EHR data to construct and validate algorithms for generalized additive models and random forest models.
The study included a total population of 58,236 patients who had 74,417 major inpatient surgical procedures. Among this group, the mean age was 57, and 50.2 percent were male. All admissions took place between June 1, 2014, and Sept. 20, 2020.
The development phase included data from 52,117 inpatient surgical procedures that took place between June 1, 2014, and Nov. 27, 2018. The validation phases included data from 22,300 inpatient surgical procedures collected between Nov. 28, 2018, and Sept. 20, 2020.
Represented in the validation cohort were 19,132 patients with a mean age of 58. Around half (50.6 percent) were male, 14.5 percent were Black, 77.2 percent were White, and 6.5 percent were of other races such as American Indian or Alaska Native, Asian, Native Hawaiian or Pacific Islander, or multiracial. Also, 5.1 percent were Hispanic, and 92.3 percent were non-Hispanic.
Researchers found that the model performance depended significantly on the number of input features, as a greater number linked to consistently strong results. Three generalized additive and random forest models were developed using 55, 101, and 135 input features. Overall, the random forest models outperformed the generalized additive ones.
Further, the random forest model trained with 135 inputs displayed the strongest abilities for predicting acute kidney injury, cardiovascular complications, neurological complications, and more. The model also achieved a predictive performance matching that of surgeons.
The researchers concluded that MySurgeryRisk, which allowed for efficient fingerprint login access and data loading at a rate faster than a web portal, has the potential to effectively support surgical decision-making.
AI and machine-learning (ML) models have been successfully applied to improve surgical care.
In April 2022, surgeons from the University of Texas MD Anderson Cancer Center in Houston created ML models to determine risks for abdominal hernia surgery complications.
These models considered patient demographics and characteristics, such as smoking status, patient outcomes, and characteristics of the procedure.
Researchers found that the ML models achieved high accuracy in predicting hernia recurrence, surgical site occurrence, and 30-day hospital readmission.