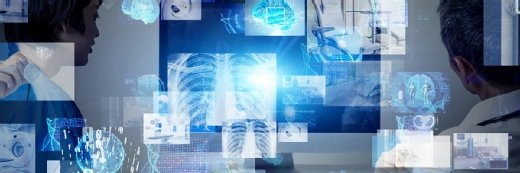
metamorworks/istock via Getty Im
AI Prediction Tool Identifies Chemotherapy Drug Toxicity Risk
A new prediction tool uses artificial intelligence and genetics to predict the risk for complications from chemotherapy drug toxicity in patients with leukemia.
Researchers from the University of Florida have created a predictive analytics tool capable of identifying acute lymphoblastic leukemia (ALL) patients’ risk for complications from chemotherapy drug toxicity, according to a study published last week in JCO Precision Oncology.
ALL is one of the most common types of leukemia in children and requires intensive chemotherapy, which can cause chemotherapy drug toxicity or adverse side effects that result from treatment. These side effects, such as heart or nerve damage, are often serious or life-threatening and result in additional disease burden, as ALL survivors are at a higher risk of chronic health conditions and early death.
“The survival rates for this type of leukemia are generally good, but one of the biggest clinical challenges for these patients is toxicity that affects their quality of life because of the intensive chemotherapy drugs they’re given,” said Jatinder Lamba, PhD, study leader and associate dean of research and graduate education and a professor in the UF College of Pharmacy, in a press release discussing the research findings. “Our goal [with this research] was to build a genetic toxicity score that can predict whether a patient has a higher or lower risk of toxicity when taking these medications.”
To generate these scores, the research team looked at toxicities in multiple categories, including neurological, gastrointestinal, and infections that resulted in hospital stays, identified within 100 days of beginning cancer treatment. Toxicity and DNA data were gathered from 75 patients treated at UF Health between 2012 and 2020.
Using these data for pharmacogenomic evaluation, the researchers demonstrated a significant association between various single-nucleotide polymorphisms (SNPs) and genes that have an impact on toxicities and help predict risk.
To further improve and refine prediction, the researchers incorporated artificial intelligence (AI) into a multivariable approach to help model combinations of up to three SNPs and other genetic variants likely to predict a particular type of toxicity. Results from this model were used to develop polygenic toxicity risk scores.
The researchers found multiple SNPs to be predictive of toxicity phenotypes in univariate analysis, and multivariable combination analysis suggested that several genes likely cause a patient’s susceptibility to chemotherapy-induced toxicity. This combination-based approach accurately predicted the most common toxicities during leukemia treatment.
The researchers posited that these results demonstrate the promise of multivariable, SNP-based models to establish clinically relevant biomarkers for toxicity risk evaluation. This may enable more personalization of cancer therapy and minimize toxicity for patients. If leveraged in the clinical setting, a risk-scoring tool like this could guide clinical decision-making and improve patient quality of life.
“With this score in a patient’s medical record, when the clinician is prescribing a particular chemotherapy, they will know the patient’s risk of getting life-threatening toxicities and be able to make informed decisions,” Lamba said. “They can watch the patient more closely or modify the drug dosages, or they can preemptively enhance supportive therapy to prevent the toxicity.”