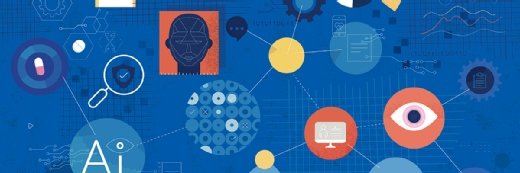
DrAfter123/DigitalVision Vectors
Artificial Intelligence Aids Psoriasis Severity Assessment
An AI-based imaging tool may help accurately assess psoriasis severity and facilitate long-term patient self-management of the disease.
Researchers have developed an artificial intelligence (AI)-based tool for the assessment of psoriasis severity in an effort to facilitate long-term chronic disease self-management for patients, according to a study published this month in the Journal of Medical Internet Research (JMIR).
According to data from the American Academy of Dermatology, roughly 7.5 million people in the United States have psoriasis. An estimated 29.5 million adults globally were thought to have the disease in 2017.
There is no cure for the chronic skin condition, but treatment can help manage symptoms. In the JMIR study, the authors noted that psoriasis could be effectively treated via teledermatology if reliable tools for psoriasis severity assessment could be developed and validated.
Currently, the Psoriasis Area and Severity Index (PASI) is one of the most common metrics for assessing severity, but the researchers stated that the tool can be highly subjective and is rarely used in clinical practice as a result.
To overcome these challenges, the research team developed an AI tool for severity assessment with the aim of facilitating the long-term management of patients with psoriasis.
The model, a deep-learning (DL) tool, was trained to estimate PASI scores using a dataset of 14,096 images from 2,367 patients 18 years and older with psoriasis who received care at a multicenter hospital from January 2015 to July 2021. Of these, images from 1,962 patients seen from January 2015 to April 2021 were used to train the model, while the remaining images from the 405 patients seen between May 2021 to July 2021 were used to validate the model. Images were captured with either a digital camera or a smartphone.
The model was designed to simulate how dermatologists calculate PASI scores in the clinical setting, using the image inputs to predict the redness of erythema, the thickness of induration, the scaling of desquamation, and the area ratio. The model also incorporated a multiview feature enhancement block, which merges features from multiple input images to help the model combine image features from different perspectives and simulate the visual diagnostic method a clinician would use.
The model was then compared with 43 experienced dermatologists to assess its accuracy.
Overall, the model outperformed the dermatologists, achieving a 33.2 percent improvement in the total PASI score, alongside 23, 7, 11, and 12 percent improvements in accuracy in the subscores for erythema, induration, desquamation, and area ratio, respectively.
The model’s ability to predict the direction of psoriasis severity progress over time was also evaluated.
The average trend accuracy was 84.81 percent, indicating the model’s ability to accurately predict the trend of the severity degree in over 80 percent of cases. Similarly, if the gap in PASI scores differed by more than five points between patient visits, meaning that the severity had changed from severe to moderate or moderate to mild, the model could predict the phenomenon with an accuracy of up to 96.09 percent.
The authors concluded that the model could automatically calculate PASI scores accurately, objectively, and efficiently. They also stated that the model’s integration into a mobile app could serve as a telehealth-based alternative to in-person psoriasis severity assessments and help support disease self-management for psoriasis patients.