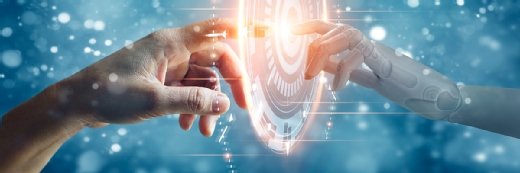
Getty Images
AI Shows Potential for Automating Hip Fractures Diagnosis
New research shows that artificial intelligence algorithms could review radiographs and accurately diagnose hip fractures, thereby automating the process.
A recent study published in JAMA Network Open found that machine-learning (ML) and artificial intelligence (AI) algorithms could use radiographs to diagnose hip fractures with comparable accuracy to expert radiologists and physicians.
Although AI has been proven to assist in the development of diagnostic tools for hip fractures, the impact and capabilities of new algorithms remain understudied, the study notes.
Using studies that focused on the development of ML models for diagnosing hip fractures from radiographs, researchers aimed to compare how the diagnostic accuracy of this technology compared to that of expert clinicians.
The criteria for study inclusion were a focus on the development of ML models for the diagnosis of hip fractures, specifically using hip or pelvic radiographs, or for predictions of postoperative patient outcomes related to surgery for hip fracture. There were 39 studies included in the analysis, 18 of which used AI models to diagnose hip fractures on plain radiographs, and 21 used AI models to predict patient outcomes after hip fracture surgery.
Across all the studies, researchers used 39,598 plain radiographs and 714,939 hip fracture radiographs to train, validate, and test models for diagnosis and postoperative outcome prediction, respectively. To evaluate the diagnostic accuracy of AI models compared to clinicians, researchers used odds ratios (ORs) with confidence intervals of 95 percent.
Researchers trained ML models to predict six postoperative outcomes: mortality, length of stay, delirium, discharge destination, hospital cost, 30-day major complications, and functional independence measure. Researchers found that mortality rates and length of stay in the hospital were the most predicted outcomes. The median accuracy of the ML models for 30-day mortality was 72.8 percent. One ML model predicted the length of stay with an accuracy of 76.5 percent.
They also noted that for hip fracture radiographs, the OR for diagnostic error of ML models was comparable to that of clinicians. Also, AI models had higher overall accuracy and outperformed clinicians regarding variability in sensitivity and specificity across models.
Based on this data, researchers concluded that using AI to support the diagnosis of hip fractures from radiographs is promising, as this technology performed similarly to radiologists and surgeons. However, the study found that the benefits of AI do not exceed those of traditional multivariable predictive statistics when it comes to predicting postoperative outcomes.
The use of AI for early diagnosis and risk prediction is growing.
In March, researchers from Rutgers, the State University of New Jersey, found that AI and ML could examine genes within DNA to assist clinicians in predicting cardiovascular diseases.
Using these models, researchers compared the genes of healthy patients and patients with cardiovascular disease and how they linked to various manifestations of the disease. This allowed them to identify a specific group of genes associated with atrial fibrillation and heart failure, as well as data regarding how various demographics contributed to these conditions.
Another study from April 2022 described how an AI system could be used for cardiac arrest predictions.
Developed by researchers from Johns Hopkins University, the AI system collected data such as the volume and mass of a patient’s heart, along with information such as age, weight, and race.
Using this data, the AI system could detect patterns that could not be observed by the naked eye, assisting researchers in predicting the probability of sudden cardiac arrest within 10 years.