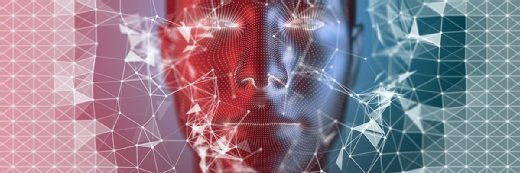
Getty Images
ML Model Uses Time, Location Data to Predict COVID-19 Transmission
A machine learning model may help predict and track COVID-19 transmission using the location and time of confirmed cases.
Researchers from Carnegie Mellon University (CMU) have developed a machine learning (ML) tool designed to predict COVID-19 transmission using location and time data, according to a study published this week in the Journal of the Royal Statistical Society.
The ability to accurately predict and track COVID-19 is key to curbing spread of the virus, and public health organizations have attempted to do so throughout the pandemic using publicly available data. These data, which often consist of the combined number of cases or deaths in a geographic area, can be biased or unreliable, the study states.
This type of data also may not adequately capture more nuanced factors that impact transmission, researchers noted.
“Most COVID-19 studies chronicle overall infection at a state or county level, reporting the aggregated number of cases in a particular region at a particular time,” explained Shixiang Zhu, PhD, coauthor of the study and assistant professor of data analytics at CMU’s Heinz College, in a press release detailing the research. “This tends to miss fine details of the virus’ propagation patterns.”
To capture these details, the CMU teamed worked with researchers from the Georgia Institute of Technology (Georgia Tech), the Universitat Jaume I in Spain, and the Universidad Nacional de Colombia to investigate COVID-19 data from Cali, Colombia, the country’s second-largest city.
The dataset, sourced from the Municipal Public Health Secretary of Cali, documents the location and time of every confirmed case in the city, rather than the combined number of cases or deaths in a geographic area.
Looking at data from March 15 to September 30, 2020, the researchers created an ML model to predict transmission using a neural network-based technique to evaluate the impact of time, location, and other spatio-temporal factors, such as population density, on virus spread.
Additionally, external influences created by the presence of city landmarks, such as town halls, schools, and churches, were included in the analysis.
Overall, the researchers found that the model successfully predicted COVID-19 transmission, suggesting that transmission correlated with socioeconomic status.
Those at increased risk of contracting the virus resided in the center, northeast, and northwest of Cali, areas where neighborhoods of low socioeconomic status (SES) were more common. Conversely, there was a low risk of contagion in the south of the city, where people of higher SES lived.
The researchers suggested that these differences may be the result of disparities in purchasing power and whether basic needs are being met in a certain population. The communities with higher risk of contagion, they explained, have more unsatisfied basic needs and significantly less purchasing power than those in the higher SES areas.
The researchers also found that the city’s landmarks played an important role in the virus’ spread.
These findings show the model’s potential to help policymakers monitor the virus, track real-time data for future epidemics, and inform health surveillance systems to support outbreak response, the researchers concluded.
“High-resolution data sets like the one we used will be more widely available in the future, so the approach we used in Cali is not limited to that jurisdiction,” said Zheng Dong, a PhD student in machine learning at Georgia Tech’s H. Milton Stewart School of Industrial and Systems Engineering, who led the study. “In fact, it can be used, extended, and adapted to several natural phenomena represented by locations in space and time.”