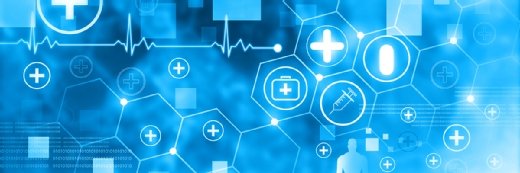
Rasi Bhadramani/istock via Getty
Machine-Learning Model Estimates US Firearm Homicides in Near Real Time
A machine-learning model can forecast US firearm homicide deaths in near real time with 99.74 percent accuracy, new research shows.
Researchers have developed a machine-learning (ML) model designed to use secondary data sources to accurately estimate the near real-time burden of weekly and annual firearm homicides in the US, according to a study published last month in JAMA Network Open.
Firearm homicides are a major public health concern in the US. Centers for Disease Control and Prevention data indicate that the firearm homicide rate increased by nearly 35 percent in 2020, reaching the highest level since 1994.
Coordinating effective public health responses to lower the firearm homicide rate is difficult because of a lack of timely mortality data, the study authors stated. To address this, they created a predictive model that could forecast firearm homicides using secondary data sources.
The researchers began by collecting anonymous, longitudinal time series data theoretically associated with firearm homicide trends and available in real time or near real time. They pulled 2014-2019 data from four online and health service sources: Google and YouTube search trends related to firearms, emergency department visits for firearm injuries per the National Syndromic Surveillance Program, and emergency medical service activations for firearm-related injuries.
Data from National Domestic Violence Hotline contacts flagged with the keyword ‘firearm’ from 2016 to 2019 were also included.
Weekly firearm homicide estimates were then calculated using a two-phase pipeline, which fit appropriate ML models to each data stream and combined the best individual models into one stacked ensemble model.
The researchers evaluated model accuracy by comparing its predictions of firearm homicides for 2019 to actual firearm homicide rates demonstrated by National Vital Statistics System death certificates. Models were also compared to a seasonal autoregressive integrated moving average (SARIMA) model, a common approach used to forecast injury mortality.
Both individual and ensemble models provided highly accurate predictions of US firearm homicides.
Ensemble models achieved higher performance than their individual counterparts in terms of mean error for weekly estimates of firearm homicides and higher annual accuracy. Using all five data sources, the ensemble model predicted the total number of firearm homicides in 2019 within 38 deaths for the entire year, with a root mean square error of 24.46 deaths and full-year accuracy of 99.74 percent.
The SARIMA model, in contrast, achieved a 95.48 percent accuracy, predicting the total number of firearm homicides within 652 deaths.
The all-source model also decreased the time lag of reporting weekly firearm homicides from seven to eight months to approximately six weeks.
These findings suggest that the all-source model has the potential to facilitate timely firearm homicide prevention efforts and expand firearm violence research using secondary data sources, the study authors concluded.
This work and other studies looking at firearm deaths come at a time when US firearm-related injuries and deaths are rising across populations.
Analyses from a June 2022 Kaiser Family Foundation (KFF) report revealed that the national suicide death rate increased 12 percent from 2010 to 2020, rising fastest among people of color and younger individuals.
The report utilized the CDC Wide-ranging ONline Data for Epidemiologic Research (WONDER) database to examine trends in suicide rates over time and by race and ethnicity, sex, age, and state.
The number of firearm-related suicide deaths remained similar to suicide by other means every year from 2010 to 2019. However, this trend changed in 2020, when rates of suicide by other means dropped by 8 percent while suicide deaths by firearm remained stable, accounting for more than half of the 45,979 suicides that year.