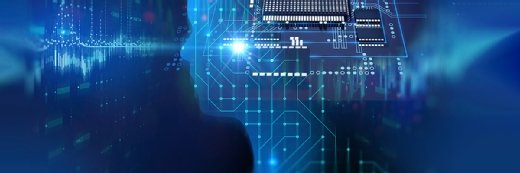
Getty Images
Wearables, ML Predict ADHD, Sleep Problems in Children
Researchers used circadian rhythm-based wearable data and a machine-learning model to predict ADHD and sleep problems in children.
A study published last month in JAMA Network Open describes how researchers combined data from wearable devices and machine-learning (ML) methodologies to help predict attention-deficit/hyperactivity disorder (ADHD) and sleep problems in children.
Statistics from the Centers for Disease Control and Prevention (CDC) indicate that ADHD is one of the most common neurodevelopmental disorders of childhood. Affected children may struggle with controlling impulsive behaviors, paying attention, or being overly active. In the US, it is estimated that ADHD affects 6 million children between the ages of three and 17 years.
The researchers noted that sleep problems often appear alongside ADHD symptoms. Both can have a significant impact on a child’s mental health. These impacts highlight the importance of early detection, but interview-based diagnostic approaches have some limitations, they stated.
To combat this, the research team developed a tool to predict ADHD and sleep problems using digital phenotypes, which were created using wearable data.
Cohort participants and their data were sourced from the National Institutes of Health (NIH)-supported Adolescent Brain Cognitive Development (ABCD) study, the largest long-term study of brain development and child health in the US.
The researchers leveraged data regarding each participant’s sex, wearable device, anthropometrics of youth, and K-SADS data from the ABCD study, expanding these to reflect participants’ daily circadian rhythms via physical biomarkers and wearable data. Wearable data was sourced from ABCD-issued mobile technologies and wearables. These data included heart rate, stage of sleep for both the 30- and 60-second records, calories, intensity, and metabolic equivalents.
To generate predictive features, the research team excluded wearable data recorded for less than 30 minutes per hour and less than five hours per day. From there, 64 circadian features were created, including bedtime and daytime heart rates and step counts, duration of naps, and duration of sleep.
Using screening data from 6,571 patients and 21 days of wearable data from 5,725 patients collected at the two-year follow-up, circadian rhythm–based features were generated for each participant. In total, 12,348 wearable data points for ADHD and 39,160 for sleep problems were merged for use in the ML models.
Of the 6,571 children with screening data, 1,090 individuals were included in the ADHD analysis, with 79 serving as true diagnoses and 1,011 as controls. Similarly, 3,414 individuals were included in the sleep problems analysis, with 68 as true diagnoses and 3,346 as controls.
For predicting each diagnosis, random forest, extreme gradient boosting, and light gradient-boosting machine (LightGBM) models were run 50 times to estimate an average performance. Performance was measured using area under the receiver operating characteristics curve, sensitivity, specificity, positive predictive value (PPV), and negative predictive value (NPV). The importance of each feature was calculated using the Shapley Additive Explanations value.
Overall, the models achieved reasonable predictive performance for ADHD, with the LightGBM model showing the highest average performance: AUC was 0.791, sensitivity was 0.718, specificity was 0.716, PPV was 0.152, and NPV was 0.973.
The LightGBM model also showed the highest average performance in the sleep problems group, with a 0.735 AUC, 0.718 sensitivity, 0.628 specificity, 0.035 PPV, and 0.992 NPV.
With regard to feature importance, the most relevant features for ADHD prediction were sex, midline estimating statistics of rhythm heart rate, maximum heart rate, daytime minimum heart rate, and duration of being asleep in a 60-second interval.
For the sleep problems group, the most important predictive features were the duration of being asleep during a nap, the duration of sedentary activity quantified using metabolic equivalents, the sum of calories, steps, and heart rate.
The researchers concluded that these findings show the potential of an ML-based predictive model for the early detection of ADHD and sleeping problems using digital phenotypes from wearables. However, follow-up studies may improve model performance further.