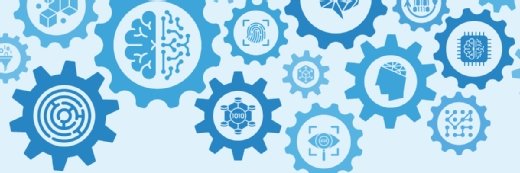
Getty Images
AI In Medical Imaging May Amplify Biases, Inequities
Researchers found that most data used to create AI algorithms from medical images do not include patient demographics, which may magnify inequities.
Researchers from the University of Maryland School of Medicine (UMSOM) found that most crowd-sourced datasets used in medical imaging data science competitions to develop artificial intelligence (AI) algorithms do not contain demographic data, and the algorithms themselves were not evaluated for inherent biases, a combination that could exacerbate care disparities.
These findings, pulled from a study published in Nature Medicine last month, indicate that researchers or clinicians who may use one of these algorithms would have no way to determine whether its training data contained representative samples of the population, or if biases were present in the data.
The findings were made following an analysis of datasets used in data science competitions, which use crowd-sourced data from around the world. Using these data, clinicians and data scientists try to develop the best, most accurate medical imaging algorithm possible.
The analysis showed that 61 percent of the data from 23 different competitions did not include demographic information such as race, age, and sex. Further, none of the competitions in the analysis had evaluations for biases against disadvantaged or underrepresented populations within algorithms.
The researchers noted that biases and inequities are already a significant issue in healthcare, as most data collected tends to skew toward people with easy access to healthcare, males, and white people. As a result, those of other races, ages, genders, and sexual orientations may not be adequately represented, which can perpetuate existing disparities.
“These deep learning models can diagnose things physicians can’t see, such as when a person might die or detect Alzheimer’s disease seven years earlier than our known tests — superhuman tasks,” explained senior investigator Paul Yi, MD, assistant professor of diagnostic radiology and nuclear medicine at UMSOM and director of the University of Maryland Medical Intelligent Imaging (UM2ii) Center, in the press release. “Because these AI machine learning techniques are so good at finding needles in a haystack, they can also define sex, gender, and age, which means these models can then use those features to make biased decisions.”
According to the press release, the US also performs more medical imaging than the rest of the world, which could cause inequities to be magnified globally if issues with bias and representation in imaging data are not addressed.
“We hope that by bringing awareness to this issue in these data competitions – and if applied in an appropriate way – that there is tremendous potential to solve these biases,” said lead author of the study Sean Garin, program coordinator at the UM2ii Center.
To combat these issues, the researchers recommended that future medical imaging data science competitions require not only high accuracy, but also fairness within each algorithm across populations.
“As AI models become more prevalent in medical imaging and other fields of medicine, it is important to identify and address potential biases that may exacerbate existing health inequities in clinical care – an essential priority for every academic medical institution,” said UMSOM Dean Mark T. Gladwin, MD, vice president for medical affairs, University of Maryland, Baltimore, and the John Z. and Akiko K. Bowers Distinguished Professor.