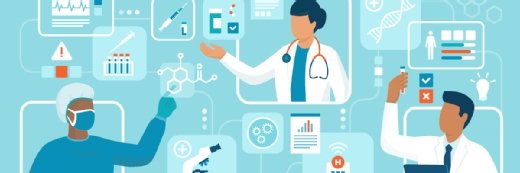
Getty Images
Johns Hopkins Machine Learning Tool Can Identify Tumor Cell Interactions
SpaceMarkers, a machine learning tool developed by Johns Hopkins researchers, may help generate insights into cancer progression and treatment.
Researchers at the Johns Hopkins Convergence Institute and the Johns Hopkins Kimmel Cancer Center have developed a machine learning (ML) model capable of identifying molecular interactions among the cells in and around tumors.
The tool, known as SpaceMarkers, leverages spatial transcriptomics, a type of technology that helps measure gene expression within a tissue sample using the genes’ locations in cells.
The press release indicates that by understanding both these intercellular interactions in the tumor microenvironment and the molecular profiles of individual cells, researchers can gain insights into tumor progression.
In the study evaluating SpaceMarkers, published last month in Cell Systems, the researchers noted that genes are expressed within each cell for a variety of reasons, but some can create higher risk for cancer progression. Highly expressed cells, for example, are associated with prominent interactions among certain cell types not typically seen in healthy tissue.
“SpaceMarkers can help cancer researchers identify if a gene is overexpressed because of cell-to-cell interactions,” explained Elana Fertig, PhD, senior author of the study who serves as the division director of oncology quantitative sciences and co-director of the Convergence Institute. “It can also identify the specific cell-to-cell interactions associated with genes of interest. This new information can provide further avenues for researchers to understand the factors responsible for cancer or answer why some patients respond to certain treatments while others do not.”
The tool is designed to analyze spatial transcriptomic data and flag regions of high activity from individual cell types. A region with high activity from more than one cell type are denoted as cell-to-cell interaction sites. From there, SpaceMarkers works to identify molecular changes that result from these interactions.
In the study, the tool was validated using clinical samples of liver, pancreatic, and breast cancers. SpaceMarkers was successful at identifying genes known to impact interactions in immune and tumor cells.
“Many genes were consistent with our existing understanding of tumor-immune interactions in these types of cancer,” said lead study author Atul Deshpande, PhD, a postdoctoral researcher in the Fertig Lab at The Johns Hopkins University. “We also found that SpaceMarkers, in its current form, is more suitable for analysis of solid tumors, such as those found in some breast and liver cancers.”
Moving forward, the researchers aim to pursue further research correlating identified genes with treatments and patient response. The research team will also work to improve SpaceMarkers’ performance on cancers that lack well-defined tumor boundaries or a distinct tumor mass, like pancreatic cancer.
This research is part of a broader trend concerned with applying artificial intelligence (AI) and ML to study tumors.
In 2021, Johns Hopkins University researchers created the first non-invasive optical technique to identify changes in tumors and responses to immunotherapy using ML.
The same year, a team from Massachusetts General Hospital (MGH) found that AI and magnetic resonance imaging (MRI) can be leveraged to detect early signs of tumor cell death following cancer therapy.