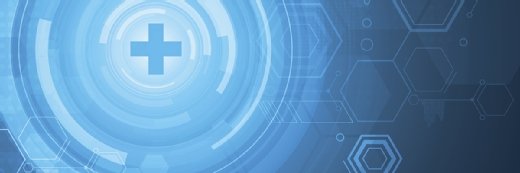
traffic_analyzer/DigitalVision V
DL Approach Enables Interpretations of Electrocardiograms as Language
A deep learning approach for electrocardiogram analysis may allow for the interpretation of ECGs as language, improving the accuracy of diagnoses.
Researchers from Mount Sinai have developed deep learning (DL)-based model for electrocardiogram (ECG) analysis that enables the interpretation of ECGs as language and may improve ECG-related diagnoses, according to a study published this week in npj Digital Medicine.
The approach, known as HeartBEiT, may be especially useful for diagnosing cardiac conditions where available data to train a model is limited.
ECGs in the United States are considered low-cost, non-invasive, and widely applicable to evaluate a patient’s heart health, but ECGs can have limited utility, the study authors explained. This phenomenon is the result of clinicians and other health professionals being unable to consistently identify patterns of disease on an ECG with the naked eye, particularly for conditions with subtle or chaotic patterns or those without established diagnostic criteria.
To address this, researchers have been investigating how artificial intelligence (AI) methods could help. The authors noted that much of this work is focused on using convolutional neural networks (CNNs) to look for patterns by sifting through large amounts of ECG data.
However, CNNs for ECG analysis require large training datasets, which are often not available. Alternatively, transformer models, which transform one type of input into another, can help combat this by leveraging an attention mechanism to define and emphasize relationships between units of input data.
These input data can be sections of an image or text, and in the study, the input data were 8.5 million ECGs from 2.1 million patients gathered over the past four decades from four hospitals within Mount Sinai’s network.
Discrete representations of small parts of these ECGs were created by the model and transformed into language, the researchers indicated. Those representations, they explained, can be conceptualized as individual words and a whole ECG as a single document.
The researchers pre-trained HeartBEiT using these data, then evaluated its performance compared to those of three CNN architectures in three cardiac diagnostic areas: hypertrophic cardiomyopathy, low left ventricular ejection fraction, and ST-elevation myocardial infarction.
Overall, HeartBEiT significantly surpassed the other models, with improved explainability of diagnosis even when it used lower sample sizes than the CNNs.
“Our model consistently outperformed convolutional neural networks,” explained Akhil Vaid, MD, first author of the study and instructor of Data-Driven and Digital Medicine (D3M) at Mount Sinai’s Icahn School of Medicine, in a press release detailing the study.
“Such CNNs are often pre-trained on publicly available images of real-world objects,” Vaid continued. “Because HeartBEiT is specialized to ECGs, it can perform as well as, if not better than, these methods using a tenth of the data. This makes ECG-based diagnosis considerably more viable, especially for rare conditions which affect fewer patients and therefore have limited data available.”
The authors emphasized that a tool like HeartBEiT is not intended to replace ECG-based diagnosis provided by clinicians, but to assist clinicians and improve outcomes. They also noted that the tool’s improved explainability may also be a boon for clinicians who wish to understand how a model generates its insights.
“Neural networks are considered black boxes, but our model was much more specific in highlighting the region of the ECG responsible for a diagnosis, such as a heart attack, which helps clinicians to better understand the underlying pathology,” said Girish Nadkarni, MD, MPH, the Irene and Dr. Arthur M. Fishberg Professor of Medicine at Icahn Mount Sinai and senior author on the study. “By comparison, the CNN explanations were vague even when they correctly identified a diagnosis.”
This research is the latest in a recent string of studies examining how deep learning could be applied to cardiovascular care.
In November, a team of researchers from Massachusetts General Hospital and Brigham and Women's Hospital developed a DL model capable of predicting a patient’s ten-year risk of death from a heart attack or stroke stemming from atherosclerotic cardiovascular disease using a single chest X-ray.
The model addresses challenges associated with current standards for evaluating the risk of major adverse cardiovascular disease events, such as missing patient variables necessary to calculate the atherosclerotic cardiovascular disease (ASCVD) risk score.
X-rays capture information beyond these variables, and the DL model provided a reliable, robust method to analyze that information.