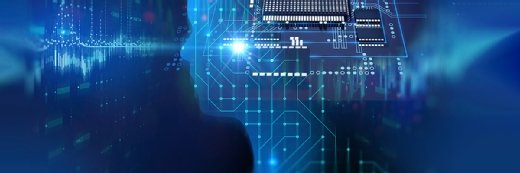
Getty Images
NYU Large Language Model Forecasts Hospital Readmissions, Length of Stay
NYUTron leverages natural language processing to successfully predict 80 percent of all-cause readmissions, a five percent improvement over standard models.
Researchers at New York University (NYU) Grossman School of Medicine have developed a large language model (LLM) capable of predicting multiple clinical outcomes, including readmissions, and the tool has been deployed across NYU Langone Health.
The model, called NYUTron, uses unaltered text from EHRs to predict 30-day all-cause readmission, in-hospital mortality, comorbidity index, length of stay, and insurance denials.
These findings were published in a study this week in Nature, in which the researchers highlighted that developing prediction models can be a challenge because most algorithms best process data that is organized and specially formatted, rather than ‘free text’ data, which is often structured similarly to how humans write and think.
The study indicates that natural language processing (NLP) algorithms can help research teams address this problem, and LLMs may help pull insights from EHRs without cumbersome data reorganization.
To test this, the researchers designed NYUTron using EHR data from 336,000 NYU Langone who received care from January 2011 to May 2020. These yielded millions of clinical notes and a 4.1 billion-word ‘language cloud’ that captured free text insights from patient progress notes, radiology reports, and discharge instructions.
Clinicians’ language within each record was not standardized to help determine the model’s ability to generate predictions without data reorganization.
Overall, the tool successfully processed these data to yield predictions, even demonstrating that it could interpret abbreviations that were unique to individual clinicians.
NYUTron accurately flagged 85 percent of patients who died in the hospital, representing a seven percent improvement over standard in-hospital mortality prediction methods. The model performed similarly on length of stay, successfully estimating 79 percent of patients’ actual length of stay, a 12 percent improvement compared to standard methods.
The model also accurately predicted the chances of an insurance denial and the presence of comorbidities.
“These results demonstrate that large language models make the development of ‘smart hospitals’ not only a possibility, but a reality,” said study senior author and neurosurgeon Eric K. Oermann, MD, in the study’s press release. “Since NYUTron reads information taken directly from the electronic health record, its predictive models can be easily built and quickly implemented through the healthcare system.”
NYUTron was designed in collaboration with NVIDIA and is fine-tuned to NYU Langone’s patient population, according to a recent post on the technology company’s blog.
“Much of the conversation around language models right now is around gargantuan, general-purpose models with billions of parameters, trained on messy datasets using hundreds or thousands of GPUs,” Oermann explained in the blog. “We’re instead using medium-sized models trained on highly refined data to accomplish healthcare-specific tasks.”
Using these refined, specific data allows the predictive model to be fine-tuned on-site using a specific hospital’s data, which helps boost predictive accuracy.
“Not all hospitals have the resources to train a large language model from scratch in-house, but they can adopt a pretrained model like NYUTron and then fine-tune it with a small sample of local data using GPUs in the cloud,” Oermann continued. “That’s within reach of almost everyone in healthcare.”