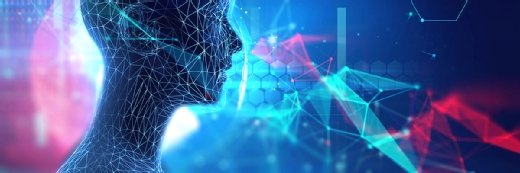
Getty Images
Dementia Risk Prediction Models May Have Limited Clinical Utility
Four common prediction scores for estimating ten-year dementia risk demonstrated limited clinical value in helping better target prevention strategies.
A study published last week in JAMA Network Open demonstrated that four widely used predictive models for estimating ten-year dementia risk have limited clinical value and utility, indicating that more accurate algorithms are needed to help target patients for dementia prevention.
Early detection and intervention for dementia patients are key to improving outcomes, but estimating dementia risk in order to inform prevention strategies remains a challenge. The researchers indicated that the clinical value of current predictive algorithms for individualized dementia risk is unclear.
To address this, the research team conducted a study to determine the clinical value of four common multifactorial algorithms used to estimate ten-year dementia risk: the Cardiovascular Risk Factors, Aging and Dementia (CAIDE-Clinical) model; the CAIDE–APOE-supplemented score; the Brief Dementia Screening Indicator (BDSI); and Australian National University Alzheimer Disease Risk Index (ANU-ADRI).
The researchers pulled data from 465,929 UK Biobank participants to evaluate each model’s performance. The study looked at patients’ dementia risk scores at baseline and ascertained incident dementia that occurred within the following ten years.
The analysis included patients with no dementia at baseline whose data contained complete information on at least one dementia risk score. Participants’ data related to hospitalizations and mortality were also pulled from linked EHRs and included.
To assess each model’s performance, the research team calculated concordance (C) statistics, detection rate, false-positive rate, and the ratio of true to false positives for each risk score. Each risk prediction algorithm was also compared to a model designed to forecast ten-year dementia risk based on age alone.
Of the 465,929 participants, 3,421 were diagnosed with dementia at follow-up.
When the threshold for a positive test result was calibrated to achieve a five percent false-positive rate, each model accurately detected only nine to 16 percent of incident dementia cases, missing anywhere between 84 and 91 percent of cases.
The model that included age only also had an 84 percent failure rate, indicating that the four risk models did not significantly outperform it.
When the threshold for a positive test result was calibrated to detect at least half of future incident dementia, the ratio of true to false positives varied. The ratio was 1 to 66 for the CAIDE–APOE-supplemented model, 1 to 116 for the ANU-ADRI, and 1 to 43 for the model based on age alone.
The findings suggest that current predictive algorithms for estimating ten-year dementia risk have limited clinical utility due to their high error rates, the researchers concluded. They recommended that more research to develop accurate algorithms be undertaken moving forward to help better target patients for dementia prevention.
This research is part of a larger effort to determine how analytics and algorithms may improve the early detection and prevention of cognitive decline.
In March, HealthITAnalytics interviewed Jared Brosch, MD, a neurologist at Indiana University (IU) Health and assistant professor of clinical neurology at IU School of Medicine, and Phyllis Ferrell, global head of external engagement for Alzheimer’s disease at Eli Lilly & Company and director of the Davos Alzheimer’s Collaborative (DAC) Healthcare System Preparedness initiative, about how a new pilot program using artificial intelligence (AI)-based digital screening tools may help flag early cognitive decline.
Brosch and Ferrell explained that the model, which aims to bolster early detection in the primary care setting through a digital clock drawing test, can capture subtle nuances that may indicate cognitive decline better than traditional screening tests. In doing so, the project aims to shift cognitive care from reactive to proactive.