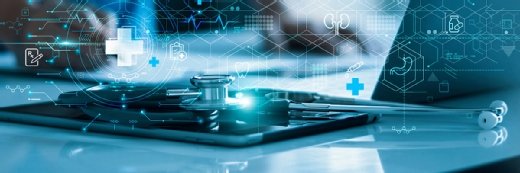
ipopba - stock.adobe.com
Generative AI May Accelerate Drug Discovery for COVID Antivirals
Oxford University and IBM have developed a new AI model that can generate antiviral molecules to target and block multiple virus proteins, including SARS-CoV-2.
Researchers have developed a generative artificial intelligence (AI) model capable of designing novel molecules to block SARS-CoV-2, the virus that causes COVID-19, according to a study published in Science Advances last week.
Drug discovery is often a long, challenging process, the researchers noted, particularly when the structure of drug-target proteins and active molecules are not known. Because of this, developing new drugs can take more than a decade.
During the COVID-19 pandemic’s early stages, research teams around the world were able to collaborate and develop new treatments for the condition significantly faster. This occurred in large part due to the fact that many of these drugs were already approved for other uses and repurposed for COVID-19.
However, the researchers indicated that in the future, new drugs may need to be developed rapidly to combat future pandemics or address mutations of existing viruses. COVID-19 has mutated several times since the onset of the pandemic, they stated, and some of the therapies developed around that time are no longer effective as a result.
This creates a unique challenge for drug discovery, necessitating an alternative method for drug discovery that is faster and more adaptable than conventional methods.
Teams at Oxford University, IBM, and United Kingdom-based synchrotron and light source facility Diamond Light Source came together to develop such an approach based on generative AI.
According to an IBM blog post discussing the research, the teams hypothesized that such an AI’s ability to process massive amounts of data and generate new insights would allow it to create entirely new molecules not found in nature that could be used to support drug discovery.
Their model, Controlled Generation of Molecules (CogMol), was built on a type of architecture called variational autoencoders (VAEs), which encode raw data into a compressed form and then translate it back into a statistical variation of the original sample, the blog post states.
The model was trained on a large dataset of molecules that were represented as strings of text, in addition to general information about proteins and how they bind.
To make the model more general and able to be deployed for molecular design tasks it has never encountered, the researchers intentionally excluded information about SARS-CoV-2 from the dataset.
From there, the model was tasked with finding drug-like molecules that would bind with two COVID-19 protein targets: the spike and the main protease, which are responsible for transmitting the virus to the host cell and helping to spread the virus.
The 3D structures of both proteins are known, but the researchers chose to use only the amino acid sequences derived from their DNA. In doing so, the researchers hoped that the model would learn to generate molecules without needing to know the shape of the protein target.
Using these amino acid sequences, CogMol generated 875,000 candidate molecules over the course of three days. These candidates were then run through multiple predictive models to narrow the number of molecules and determine what ingredients would be required to synthesize them.
From there, 100 molecules per target were selected, and chemists chose the four molecules from each target that would be easiest to manufacture.
These eight molecules were then synthesized into compounds and tested in target inhibition and live virus neutralization tests. Two of these targeted the main protease, and another two targeted the spike protein. The latter compounds proved capable of neutralizing all six major COVID-19 variants.
These findings are important, the researchers explained, because preparation for a future pandemic requires the ability to quickly develop drugs that target different sites of the protein, which is part of how a virus is neutralized.
“We created valid antivirals using a generative foundation model that knew relatively little about its protein targets,” said the study’s co-senior author, Jason Crain, PhD, a researcher at IBM Research and professor at Oxford, in the blog post. “I’m hopeful that these methods will allow us to create antivirals and other urgently needed compounds much faster and more inexpensively in the future.”
Now that the technology to generate these compounds has been developed, the researchers posited that it could potentially be used to address the threat of new viruses quickly.
“It took time to develop and validate these methods, but now that we have a working pipeline in place, we can generate results much faster,” said study co-senior author, Payel Das, PhD, a researcher at IBM Research. “When the next virus emerges, generative AI could be pivotal in the search for new treatments.”
This research is a significant example of how COVID-19 can help refine AI in healthcare.
The pandemic helped shed light on some of healthcare’s biggest shortcomings, including clinician burnout, care gaps, and disparate IT systems, forcing health system leadership to consider solutions to these problems beyond COVID-19.
For many, this meant turning to AI and data analytics tools for various use cases, including medication adherence, medical imaging, chronic disease management, clinical decision support, cancer care, and precision medicine.
While some of these use cases have a ways to go before being widely deployed in the clinical setting, stakeholders are hopeful that these technologies will transform healthcare.