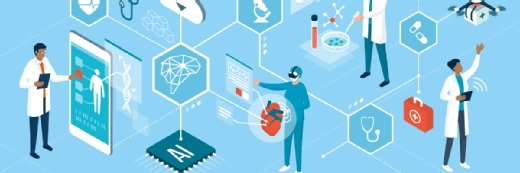
Getty Images
Blood Test-Based Model Accurately Predicts Lung Cancer Death Risk
When combined with a blood test, a personalized risk model outperforms USPSTF criteria for identifying those at high risk of lung cancer mortality.
Researchers at the University of Texas MD Anderson Cancer Center have developed a predictive tool that combines a curated blood test and a personalized risk model to flag those at high risk of dying from lung cancer better than current United States Preventive Services Task Force (USPSTF) criteria.
The combination model, described in a study published in the Journal of Clinical Oncology last week, leverages a blood-based four-protein panel (4MP) recently developed by MD Anderson researchers. By combining the blood test with a risk model known as PLCOm2012, the research team aims to lower death rates by improving lung cancer screening.
“This simple blood test has the potential to save lives by determining the need for lung cancer screening on a personalized basis,” said co-corresponding author Samir Hanash, MD, PhD, professor of Clinical Cancer Prevention at MD Anderson Cancer Center, in the press release. “Given the challenges associated with CT as a frontline screening method for lung cancer and the fact that most individuals diagnosed with the disease do not meet current [USPSTF] guidelines, there is an urgent demand for an alternative approach."
The USPSTF currently recommends that adults aged 50 to 80 years who have a 20 pack-year (PY) smoking history and who currently smoke or have quit within the past 15 years undergo annual screening via low-dose CT scan.
This recommendation is an update to previous criteria from 2013 that suggested screening for those aged 55 to 80 years with a 30 PY smoking history, a change that researchers have noted may significantly reduce lung cancer deaths.
However, the American Lung Association reports that lung cancer is the leading cause of cancer death in the US, resulting in 23 percent of all cancer deaths in 2019. This high mortality rate has led some research teams, including those at MD Anderson, to investigate how screening may be improved.
In this study, the researchers leveraged pre-diagnostic blood samples from the Prostate, Lung, Colorectal and Ovarian (PLCO) Cancer Screening Trial. This yielded a study cohort of 552 individuals who later developed lung cancer and 2,193 who did not.
Of those diagnosed with lung cancer over the six-year study period, 70 percent died from the disease.
The research team investigated the relationship between risk scores generated by the combination model and the incidence of lung cancer death within the cohort.
From there, the researchers assessed the model’s ability to accurately predict lung cancer mortality. They found that the 4MP/PLCOm2012 combined model had better sensitivity, specificity, and positive predictive value than both the 2013 and 2021 USPSTF criteria among individuals with at least a ten PY smoking history.
These results demonstrate that such a tool could improve patient outcomes, the research team indicated.
“For individuals who currently are not eligible for lung cancer screening, a positive test may help to identify those possibly at risk for lung cancer death,” said co-corresponding author Edwin Ostrin, MD, PhD, assistant professor of General Internal Medicine at MD Anderson. “We envision this as a tool that could be deployed worldwide, as the future of early detection of this disease.”
Other approaches are also being developed to tackle lung cancer risk.
In January, researchers from Mass General Brigham and the Massachusetts Institute of Technology (MIT) created a deep learning (DL) tool to predict six-year lung cancer risk among individuals with or without a significant smoking history.
The research team noted that an increase in lung cancer rates among non-smokers, in combination with the fact that current USPSTF guidelines do not provide screening recommendations for this population, necessitates the development of broader screening strategies.
To this end, they developed Sybil, a tool capable of analyzing low-dose CT scans and predicting lung cancer risk across populations. The tool relies on only one scan to do so, not requiring additional clinical data or radiologist annotations to generate its predictions.
The researchers hope that Sybil may facilitate real-time, point-of-care clinical decision support in the future.