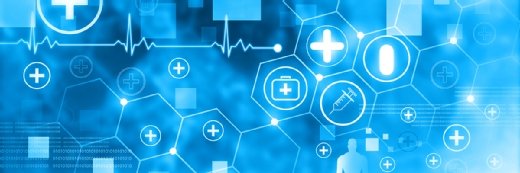
Rasi Bhadramani/istock via Getty
Machine Learning Accurately Triages Respiratory Symptoms in Primary Care
A machine learning tool can effectively categorize patients with respiratory symptoms into risk groups prior to a primary care visit, which may improve triage.
Researchers have demonstrated that a machine learning (ML)-based clinical decision support tool can accurately triage patients with respiratory symptoms before they visit a primary care clinic, which may improve care and clinician workloads, according to a study published recently in The Annals of Family Medicine.
The researchers indicated that respiratory symptom complaints are one of the most common in primary care settings. These symptoms often resolve on their own, but some indicate a more severe illness or serious condition, making effective triage of patients with respiratory symptoms crucial.
However, the research team noted that increasing healthcare costs and clinician workloads make triage a challenge. To combat this, they trained an ML model to triage patients with respiratory symptoms before these patients visit a primary care clinic.
The researchers explained that triaging patients prior to an in-person primary care consultation may prove useful by differentiating between high- and low-risk individuals, reducing the number of unnecessary interventions, such as chest X-rays and prescriptions, for those who do not need them.
The research team trained the model using clinical features and questions that would typically be asked before a primary care visit.
The researchers also extracted clinical text notes from 1,500 medical records of patients in Reykjavik, Iceland that received one of seven International Classification of Diseases 10th Revision (ICD-10) codes: J00 (common cold), J10 and J11 (influenza), J15 (bacterial pneumonia), J20 (acute bronchitis), J44 (chronic obstructive pulmonary disease [COPD]), and J45 (asthma).
Information extracted from clinical notes included data related to prescription rates, imaging referrals, and the clinician’s interpretation of each patient's symptoms.
Using these data, patients were categorized into five diagnostic groups. From these groups, patients were then sorted into ten risk subgroups based on their probability of a lower respiratory tract infection diagnosis.
Overall, the researchers found that patients in risk subgroups one through five were younger, with lower rates of antibiotic prescription, re-evaluation in primary and emergency care, chest X-ray referrals, and chest X-rays with signs of pneumonia.
Those in these subgroups actually had no chest X-rays with signs of pneumonia or a pneumonia diagnosis from their clinician.
Groups six through ten were considered high-risk, and these patients had higher rates of symptoms like lung inflammation.
The researchers concluded that these findings show how an ML model could help reduce the number of unnecessary interventions, such as chest X-rays, in low-risk groups. This would decrease the number of clinically insignificant, incidental findings without placing additional burdens on clinicians or increasing costs for patients.
This research is part of a growing area of study looking at how artificial intelligence (AI) and ML can be used to improve triage.
In January, researchers highlighted how a deep learning (DL) model could help improve care for patients who present at the hospital with acute chest pain.
The tool uses chest X-rays to flag individuals with these symptoms who may need immediate medical attention, as acute chest pain can have serious causes, such as acute coronary syndrome, pulmonary embolism, or aortic dissection.
Clinical tests to detect and diagnose acute chest pain syndrome are plagued by low specificity, leading clinicians to turn to pulmonary and cardiovascular diagnostic imaging. However, these tests can create inefficiencies in chest pain triage.
Researchers aim to streamline and improve this process through deep learning and other advanced technologies.