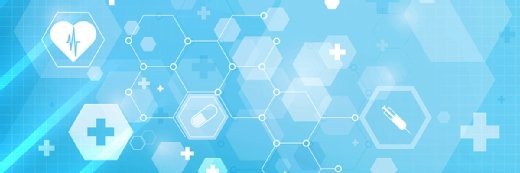
Rifqyhsn Design/istock via Getty
Machine Learning Tools Flag Predictors of Fetal Heart Rate Changes
New machine learning approaches may help identify crucial factors that can forecast fetal heart rate changes after neuraxial analgesia in pregnant patients.
Researchers have developed machine learning (ML) methods that can accurately identify predictors associated with fetal heart rate changes following neuraxial analgesia in healthy pregnant patients, according to a study published last month in BMC Pregnancy and Childbirth.
The researchers explained that neuraxial analgesia, a widely-used labor analgesia method in the United States that includes spinal, epidural, and combined spinal-epidural (CSE) techniques, is associated with fetal heart rate changes.
These changes can resolve on their own, but a significant drop in heart rate, known as fetal bradycardia, can indicate potentially serious health problems. However, the research team further noted that multiple factors can increase the likelihood of fetal bradycardia, making the condition difficult to predict.
To address this, the researchers turned to ML. A condition with several possible predictor variables like fetal bradycardia requires analyzing large amounts of data to identify which predictors are most useful in the clinical setting.
In a blog post discussing their research, the study’s authors indicated that the dynamic nature of ML models makes them well-suited to such a task.
“These models are particularly useful for managing multiple predictor variables with countless potential interactions, which may require more work to account for using traditional models,” the authors write. “The algorithms used in this branch of artificial intelligence [AI] incorporate predictor variables that may not be visible by mere background knowledge. Furthermore, when unsupervised machine learning methods are used, unknown patterns can be unveiled.”
ML algorithms also don’t make assumptions about the relationship between a predictor and an outcome, whereas humans are more likely to assume the two have a linear relationship, the authors explained. This can lead to improved accuracy among ML algorithms.
To design models appropriate for identifying predictors of fetal heart rate changes, the researchers conducted a retrospective analysis of 1,077 healthy laboring patients who received neuraxial analgesia.
Using these data, the research team compared the performance of four models: principal components regression, random forest, elastic net model, and multiple linear regression. For each model, prediction accuracy and interpretability were evaluated.
The researchers found that the random forest model achieved the best performance in terms of accuracy using mean squared error (MSE), a measure that represents the average difference between predicted and measured values.
The random forest model had an MSE of 0.9, while the other three models had MSEs of 42 or higher.
The analysis also showed that multiple factors play a significant role in fetal heart rate changes after neuraxial labor analgesia, including the mother’s body mass index (BMI), the length of the first stage of labor, the use of CSE techniques for neuraxial analgesia, and the amount of bupivacaine administered.
The researchers stated that these findings have key practical implications in shedding light on poorly understood medical problems. Within the context of their study, they noted that an ML approach has the potential to increase clinicians’ awareness of risks related to fetal heart rate changes in healthy pregnant patients, which would allow them to adjust treatment plans.
Other researchers and clinicians are also seeking to leverage AI to improve labor and delivery outcomes.
Last year, Mayo Clinic researchers developed an AI-based risk prediction model to forecast pregnant patients’ individual labor risks associated with vaginal delivery.
The model uses patient data gathered from patients’ most recent clinical assessments, cumulative labor progress from admission, and baseline characteristics at the start of labor to indicate potential delivery outcomes for the patient and their baby.
The researchers indicated that the model will be validated within labor units at Mayo Clinic.