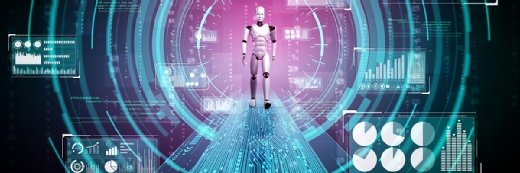
Getty Images
Deep Learning Model Detects Diabetes Using Routine Chest Radiographs
New deep learning tool leverages chest x-rays and electronic health record data to flag elevated diabetes risk up to three years before diagnosis.
Researchers at Emory University have validated a deep learning (DL) model capable of detecting early warning signs of diabetes using routinely collected chest radiographs and electronic health record data (EHR) in patients who do not meet the guidelines for elevated risk.
The tool may bolster early detection of the disease and help providers prevent diabetes-related complications. In the study describing the model, the researchers noted that the ability to flag early signs of the disease could have significant positive impacts on care, as diabetes prevalence in the United States has risen dramatically in recent decades.
As of 2021, the United States Preventive Services Task Force (USPSTF) recommends that asymptomatic adults aged 35 to 70 years who have overweight or obesity should be screened for prediabetes and type 2 diabetes.
However, the researchers indicated that this screening strategy may miss a large number of at-risk patients, especially for patients of various racial and ethnic groups for whom body mass index (BMI) is a less effective diabetes risk predictor.
Undiagnosed diabetes can lead to a host of complications and adverse outcomes, including heart disease, kidney disease, blindness, and death.
However, many people have chest x-rays each year for various reasons, and these images become part of a patient’s EHR. The advent of health AI has led to much research into how the technology may change medical imaging, and these studies have revealed that clues to many diseases can be found in radiographs.
These images, stored in the patient’s EHR, could then be analyzed for insights into the presence of conditions like diabetes.
“Chest x-rays provide an ‘opportunistic’ alternative to universal diabetes testing,” said Judy Wawira Gichoya, MD, assistant professor of radiology and imaging sciences at Emory who helped lead the study, in the press release detailing the findings. “This is an exciting potential application of AI to pull out data from tests used for other reasons and positively impact patient care.”
To develop their model, the researchers trained a DL algorithm to flag features that best predicted future diabetes diagnosis and provide a risk score using 270,000 x-ray images from a retrospective cohort of 160,000 patients. Because the approach is unlike most standard methods to forecast diabetes risk, the research team also relied on explainable artificial intelligence (AI) techniques to understand how and why the model reached its conclusions.
They found that the location of fatty tissue visible in chest x-rays plays a key role in predicting diabetes risk. The researchers further explained that this finding is in line with recent research suggesting that the presence of visceral fat in the upper body and abdomen is linked to hypertension, insulin resistance, and type 2 diabetes.
The team at Emory then externally validated the model using chest x-ray data from 10,000 patients, finding that the tool predicted diabetes risk better than a model using only non-image clinical data. In addition, the model was capable of identifying high diabetes risk up to three years prior to a patient’s official diagnosis.
The researchers posited that the model’s risk scores could help providers personalize treatment approaches for their patients.
“Diabetes is a chronic disease where body fat distribution matters. The longer the duration of the disease and the worse the glycemic control, the higher the risk of complications,” explained Francisco Pasquel, MD, associate professor in Emory’s Division of Endocrinology, Metabolism and Lipids, in the press release. “The opportunistic approach of using chest x-rays to identify those at the highest risk of diabetes, even before a spike or drop in blood sugar levels occurs, is a promising method that may help improve outcomes through early preventive measures or treatment.”
Moving forward, the research team will investigate how to further validate the tool and incorporate it into EHRs to help encourage care teams to pursue diabetes screening for patients who do not fit the USPSTF guidelines.
Other researchers are also exploring how AI can help predict chronic diseases.
In 2020, a team from MIT’s Computer Science and Artificial Intelligence Laboratory (CSAIL) shared that researchers had developed a machine learning (ML) model capable of forecasting heart failure risk using a single x-ray.
The tool is designed to detect the severity of excess fluid in patients’ lungs, which is often an early sign of heart failure that dictates treatment course. Determining these fluid levels can be a challenge, however, leading providers to utilize subtle x-ray features to give diagnoses and guide treatment.
The model quantifies fluid severity on a four-point scale, from zero (healthy) to three (very bad). The tool determined the correct level of excess fluid in over half of all cases, and it correctly diagnosed level three cases 90 percent of the time.