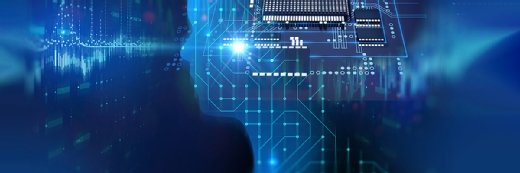
Getty Images
Experts: Medical Community Must Help Shape Use of LLMs in Healthcare
With interest in large language model applications growing, researchers argue that the medical community must help guide how these tools are used in healthcare.
In a special communication published this month in JAMA, researchers discussed the creation and adoption of large language models (LLMs) in healthcare, asserting that the process must be actively shaped by the medical community.
The authors indicated that some healthcare stakeholders are leveraging off-the-shelf LLMs developed by technology companies in an effort to determine how these tools can reshape medicine. However, the researchers proposed the opposite approach: examining how the intended medical use of an LLM or chatbot could shape their development and training.
“[B]y simply wondering how the LLMs and the applications powered by them will reshape medicine instead of getting actively involved, the agency in shaping how these tools can be used in medicine is lost,” they wrote, noting that LLM-based applications in healthcare are being deployed without being trained on medical records and without verifying the potential benefit of their use.
To combat this, the researchers stated that the medical community needs to guide the creation and deployment of healthcare LLMs “by provisioning relevant training data, specifying the desired benefits, and evaluating the benefits via testing in real-world deployments.”
Doing so requires healthcare stakeholders to ask two questions: “Are the LLMs being trained with the relevant data and the right kind of self-supervision?” and “Are the purported value propositions of using LLMs in medicine being verified?”
The authors explained that LLMs work by learning the probabilities of occurrence for particular sequences of words from a body of text, akin to autocomplete tools, to predict the next word in a sentence. These probabilities are learned using bodies of text with trillions of words, resulting in a model with billions of potential parameters.
This process also enables additional LLM capabilities, including the ability to summarize text or answer questions, without explicitly training the model for those tasks. These capabilities allow LLMs to perform certain healthcare tasks, such as summarizing medical dialogues, responding to patient queries, writing histories and physical assessments, simplifying radiology reports, extracting drug names from clinical notes, and passing the United States Medical Licensing Exam (USMLE).
The authors noted that the development of LLMs capable of these tasks relies on the ability to learn useful patterns within massive unlabeled datasets using self-supervision, in addition to the tuning of an LLM to generate responses in line with human expectations through the process of instruction tuning.
General-purpose LLMs are built and trained to these ends, meaning that they can adequately perform many medically relevant tasks. However, the researchers highlighted that these models are not exposed to medical records during their self-supervised training, and few are instruction tuned for medical tasks.
“By not asking how the intended medical use can shape the training of LLMs and the chatbots or other applications they power, technology companies are deciding what is right for medicine,” the authors alleged. Further, they stated that the medical community has mistakenly allowed technology companies and other stakeholders to take an outsized role in guiding the creation, design, and adoption of health information technology (IT) systems in the past.
Given the significant potential of advanced technologies to improve healthcare, the same mistake cannot be made with regard to LLMs, they posited.
By asking whether LLMs are being trained using relevant data and appropriate self-supervision, the medical community can play an important role in model creation by shaping instruction tuning. The authors recommended that healthcare stakeholders discuss how to create shared instruction tuning datasets and examples of LLM prompts to be fulfilled.
The researchers also suggested that health systems train shared, open-source models using their own data. Technology companies should also be queried as to whether their LLMs were exposed to any medical data while training and whether the self-supervision approach used to train the model is relevant for the healthcare use case in question.
By investigating whether the potential value propositions of LLMs in medicine have been verified, the authors stated that medical stakeholders can better quantify the benefit of each model’s use.
They noted that current LLM assessments do not effectively capture or quantify the potential benefits of human-model collaboration, which is critical to the deployment and use of LLMs in the healthcare setting.
The researchers further underscored concerns like training dataset contamination and the phenomenon of using standardized examinations designed for humans to evaluate a model.
They illustrated this point through the analogy of a human applying for a driver’s license. In this scenario, the human is assessed using a multiple-choice, knowledge-based test, while the car is subject to government- and relevant stakeholder-based regulations during the manufacturing process to ensure safety. When a car is deemed safe, the human uses it to complete their road test and become certified to drive.
The car, however, does not take a multiple-choice test or get a driver’s license, but that logic is applied when people assert that an LLM can give medical advice because it passed a medical licensing exam, the authors indicated.
With this in mind, the benefits of technologies like LLMs must be defined, and appropriate evaluations to verify those benefits must be conducted, the researchers noted. These evaluations would also help to clarify the medical-legal risks of LLM use in healthcare and inform strategies to address LLM hallucination.
Balancing the creation of healthcare LLMs with verifications of their presumed value propositions is crucial to successfully leveraging them to augment clinicians’ judgment, the authors concluded.
These warnings around the potential pitfalls of healthcare LLMs come as health systems are already deploying them for various use cases.
In June, New York University (NYU) Grossman School of Medicine researchers shared that they had created an LLM, known as NYUTron, capable of predicting various clinical outcomes.
The tool uses natural language processing to extract relevant EHR data and predict 30-day all-cause readmission, in-hospital mortality, comorbidity index, length of stay, and insurance denials.
NYUTron achieved a five percent improvement in predicting readmissions over standard models, and it has since been deployed across NYU Langone Health.