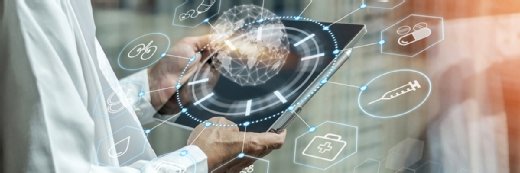
Chinnapong - stock.adobe.com
Machine Learning Predicts Esophageal, Stomach Cancers Using EHR Data
A new machine learning tool accurately predicts incident esophageal adenocarcinoma and gastric cardia adenocarcinoma three years prior to diagnosis using EHR data.
A machine learning (ML) model can automatically predict esophageal adenocarcinoma (EAC) and gastric cardia adenocarcinoma (GCA) three years before a formal diagnosis, according to a study published recently in Gastroenterology.
The researchers indicated that EAC and GCA rates have risen significantly in the United States and other western countries over the past fifty years, facilitating the need for improved screening.
“Screening can identify pre-cancerous changes in patients, Barrett’s esophagus, which is sometimes diagnosed in individuals who have long-term gastroesophageal reflux disease, or GERD,” explained Joel Rubenstein, MD, a professor of internal medicine at Michigan Medicine and a research scientist at the Lieutenant Colonel Charles S. Kettles Veterans Affairs Center for Clinical Management Research, in a press release detailing the research.
Early detection of these cancers can help patients and care teams take steps to prevent progression, but many providers aren’t aware of the relevant screening guidelines. As a result, many patients eventually diagnosed with EAC or GCA are found to have never undergone any screening, he continued.
To help address this, the research team sought to build an automated tool that could be embedded into EHRs to help guide clinical decision-making.
The resulting model, the Kettles Esophageal and Cardia Adenocarcinoma predictioN (K-ECAN), was trained using data from the Veterans Health Administration (VHA) Corporate Data Warehouse. From there, 8,430 patients diagnosed with EAC and 2,965 with GCA were identified in the VHA Central Cancer Registry and compared to 10,256,887 controls.
“K-ECAN uses basic information already readily available in the EHR, like patient demographics, weight, previous diagnoses and routine laboratory results, to determine an individual’s risk of developing esophageal adenocarcinoma and gastric cardia adenocarcinoma,” said Rubenstein.
This can help providers better determine each patient’s risk.
“Symptoms of GERD, like heartburn, are an important risk factor for esophageal adenocarcinoma,” Rubenstein stated. “But most people with GERD symptoms will never develop esophageal adenocarcinoma and gastric cardia adenocarcinoma. In addition, roughly half of the patients with this form of cancer never experienced prior GERD symptoms at all. This makes K-ECAN particularly useful because it can identify people who are at elevated risk, regardless of whether they have GERD symptoms or not.”
The model was found to have better discrimination than existing guidelines and two previously validated prediction models, and Rubenstein noted that K-ECAN can “accurately predict cancer at least three years prior to a diagnosis.”
The research team indicated that incorporating a tool like K-ECAN into EHRs could help alert providers regarding which patients are at risk of EAC or GCA via an automatic notification at opportune times, such as when the patient is due for a colorectal screening.
Doing so, they suggested, could significantly decrease the burden of these cancers.
Moving forward, the researchers will work to validate K-ECAN outside of the VHA.
Other efforts are also underway to improve cancer care using ML.
Earlier this month, researchers externally validated an ML model developed to predict six-month mortality risk for patients with advanced cancer initiating a new line of therapy (LOT).
The model was originally built to classify patients’ mortality risk to help facilitate serious illness conversations between providers and patients at various treatment decision points (TDPs).
The model uses 45 features gathered from EHRs that are implementable via the Fast Healthcare Interoperability Resources (FHIR) standard and was found to accurately categorize patient mortality risk, highlighting the potential for these tools in oncology care.