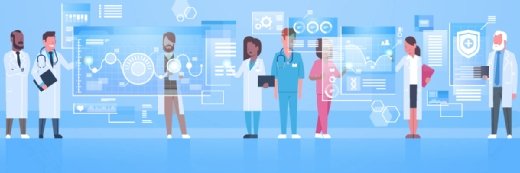
mast3r - stock.adobe.com
ML-Based Automated Screening Tool Can Determine Pulmonary Fibrosis Risk
A new machine learning-based screening tool for EMRs can accurately identify patients who have or are at high risk of developing idiopathic pulmonary fibrosis.
Researchers from Weill Cornell Medicine, NewYork-Presbyterian, the University of Chicago, Brigham and Women’s Hospital, and Mayo Clinic have created a machine learning (ML)-based screening tool integrated into EMRs that can effectively identify those at high risk of idiopathic pulmonary fibrosis (IPF) before symptoms arise.
According to the National Library of Medicine’s MedlinePlus, IPF is a chronic, progressive lung disease characterized by a buildup of scar tissue in the lungs. The disease can lead to other serious lung conditions, such as lung cancer, pulmonary embolism, pulmonary hypertension, and pneumonia. In the US, approximately 100,000 people have IPF, with 30,000 to 40,000 new cases diagnosed annually.
The disease is considered fatal, with most patients surviving only three to five years after diagnosis. According to the researchers who developed the new ML tool, IPF is generally diagnosed once symptoms are recognized, which is typically later in the disease’s course. Treatments are usually less effective at this stage, making earlier risk identification and detection of IPF crucial to improve health outcomes.
Diagnosis can also be challenging because it requires a multidisciplinary team of pulmonary clinicians, radiologists, and laboratory and pathology specialists, alongside lab tests, the press release notes.
The new screening tool is designed to streamline this process, detecting IPF risk automatically in primary care settings using information from a patient's EHR, according to a study conducted by the tool's creators. If implemented in clinical care, the tool would flag these high-risk patients for the primary care physician, who would then refer the patient for additional screening and lab tests.
“This tool takes literally zero additional minutes [of patients’ time] and can recognize features of the disease before symptoms manifest,” said study co-author Ishanu Chattopadhyay, PhD, an assistant professor of medicine at the University of Chicago, in the press release. “As soon as someone schedules a visit with their primary care physician, the program can run the screening tool and get the results before the patient even walks into the clinic.”
The team trained the tool using US-based commercially available insurance claims data from 2003 to 2018. They then validated the algorithm using three other claims datasets. Overall, the model was developed using 3 million patient records, including 54,000 IPF cases, according to the press release.
During validation, the researchers found that the tool achieved high performance in terms of both specificity and sensitivity in identifying high-risk patients. Compared with unscreened patients, those in the study with risk scores above the detection threshold had more than 30 times the chance of receiving an IPF diagnosis during the following year.
Moving forward, the research team hopes to see the tool evaluated in clinical trials and real-world primary care settings, potentially shifting IPF screening approaches in the future. The researchers also indicated that similar models and methods might be useful for other conditions where early detection could significantly improve outcomes.
This research is part of larger efforts to address pulmonary fibrosis using data analytics and AI.
This month, HealthITAnalytics interviewed Junelle Speller, vice president of the Pulmonary Fibrosis Foundation (PFF) Registry, to discuss how leveraging self-reported patient data can support clinical care and research for pulmonary fibrosis and other interstitial lung diseases.
In 2019, the National Institutes of Health and Three Lakes Partners awarded a $22 million grant to support the launch of the PRECISIONS (Prospective tReatment EffiCacy in IPF uSing genOtype for Nac Selection) study, which aims to transform the diagnosis and treatment of IPF through precision medicine.