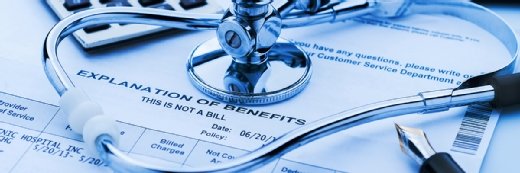
Christian Delbert - stock.adobe.
Clinical Trials Assessing ML Methods Lack Transparent Reporting, Inclusivity
Researchers found several areas of concern within randomized clinical trials examining machine-learning interventions, including noncompliance with reporting guidelines and risk of bias.
A study published in JAMA Network Open found that researchers must improve the randomized clinical trials (RCTs) to test machine-learning (ML) algorithms by making the trials more inclusive and improving reporting transparency.
Despite the potential of ML to enhance patient care, only a few RCTs have been conducted to test ML methods, researchers found.
For this study, researchers gathered past RCTs and reviewed aspects such as design, reporting standards, risk of bias, and inclusivity. They did this through a literature search that gathered 19,737 articles, of which 41 RCTs had a median of 294 participants. Of this sample of RCTs, 16 occurred in 2021, 21 took place at single sites, and 15 involved endoscopies.
To examine RCT transparency and reproducibility, researchers assessed each trial's adherence to CONSORT-AI, a reporting guideline for AI intervention trials. No RCT met all the CONSORT-AI criteria. The most common reasons cited for noncompliance were not reviewing unavailable input data (93 percent), failing to analyze performance errors (93 percent), and the absence of a statement about code or algorithm availability (90 percent).
Further, the overall risk of bias was high in 17 percent of the trials studied, and only 27 percent of trials reported race and ethnicity data. The median proportion of participants from minority groups with limited representation was 21 percent in the trials that did report race-related data.
Thus, researchers concluded that although machine learning-based algorithms are being developed, a limited number of them undergo RCTs. In addition, many existing RCTs contain high variability in adherence to reporting standards and risk of bias.
Ultimately, the study concluded that these issues must be addressed as RCT efforts continue.
But researchers also noted several limitations to the study, including that RCTs selected for the study only assessed machine-learning interventions directly impacting clinical decision-making. Also, the data collected for the analysis may no longer be considered current due to rapidly evolving research in this area.
Previous studies have gathered similar findings regarding the need for and optimization of RCTs.
In a systematic review published in August, researchers found that there are limited numbers of RCTs that evaluate artificial intelligence (AI), which are necessary to optimize the future of AI in clinical care.
Research from March found that machine learning was valuable in linking age and intensive care use to risk for pressure ulcers. But they also noted that using an RCT would have been valuable for the study.
In May, the Patient-Centered Outcomes Researcher Institute (PCORI) dedicated $262 million in funding to research focused on postpartum care and hypertension management, among other clinical areas. This grant included $15 million for researchers who studied RCTs and how they could improve care for hypertension among people who faced health disparities.