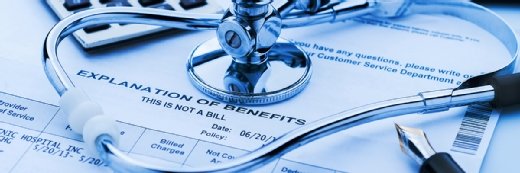
Christian Delbert - stock.adobe.
Artificial Intelligence Flags Knee Osteoarthritis Using Medical Images
New AI model helped diagnose knee osteoarthritis in 178% more cases than currently diagnosed in a large patient cohort using only X-rays of the knee joint.
Researchers from the University of Texas at Austin’s (UT Austin) College of Natural Sciences and Dell Medical School have developed an artificial intelligence (AI) model capable of identifying knee osteoarthritis based on X-ray images of the knee joint.
The work, detailed in a recent npj Digital Medicine study, underscores that knee osteoarthritis is a major cause of adult disability in the United States, particularly in patients over the age of 50. The condition can make everyday activities like standing, walking, or climbing stairs challenging or nearly impossible, and once it becomes severe, knee osteoarthritis can become costly to treat.
Arthritis of the knee is caused by the grinding of the femur and the tibia against one another, which causes the protective cartilage to wear down. This wearing down creates a gap between the two bones, known as the joint space.
The size of the joint space is associated with arthritis severity, leading clinicians to leverage it as a clinical biomarker to assess the disease in the clinical setting.
This led the research team to hypothesize that accurately evaluating the joint space, as presented in X-ray images of the knee joint, using AI could help improve diagnosis, disease progression monitoring, and potentially predict knee osteoarthritis risk.
“Imagine someone goes into the clinic and gets an X-ray for some other reason, and the clinic is running this AI system in the background on every image that automatically diagnoses arthritis,” said Vagheesh Narasimhan, PhD, an assistant professor in UT Austin’s Department of Statistics and Data Sciences and Department of Integrative Biology, in the press release. “We could tell them, perhaps you should consult an orthopedic expert about this condition.”
To design the model, the researchers pulled electronic health record data, including genetic information and medical imaging, from 29,257 individuals in the UK Biobank, a large-scale biomedical research database comprised of health information from half a million participants in the United Kingdom.
From there, the research team trained a deep learning (DL) algorithm to analyze paired dual energy X-ray absorptiometry (DXA) imaging and genome sequence data. The researchers then trained a second DL algorithm to measure the width of the knee joint space, a quantitative phenotype associated with knee osteoarthritis severity.
These could then be combined to identify disease cases.
The resulting model demonstrated clinician-level performance, accurately flagging 178 percent more cases of knee osteoarthritis than those currently diagnosed in the patient cohort.
Additionally, patients identified by the model reported higher rates of knee pain, for longer durations, and with increased severity compared to patients in the control group.
Knee osteoarthritis’ potential to cause disability and other adverse outcomes further spurred the research team to design the AI tool as a clinical decision support tool that could be incorporated into existing software leveraged by technicians to assess medical imaging.
Doing so may help predict who may be at risk for the condition and enhance early detection, the research team indicated.
“So we’re also thinking about ways to combine imaging, genetic data and other risk factors simultaneously into a single model, to provide a more comprehensive prediction for knee osteoarthritis,” Narasimhan explained.
This research reflects a broader interest in how AI can be utilized to advance medical imaging.
Last week, Microsoft and healthcare technology company Paige announced a collaboration aimed at developing the world’s largest image-based AI models for oncology and pathology.
The partnership is working to build an AI model trained using billions of imaging parameters and other data in the hopes of capturing subtle features within cancer imaging.
In doing so, stakeholders suggest that the model has the potential to significantly advance oncology and pathology, leading to transformations in cancer diagnosis and care.