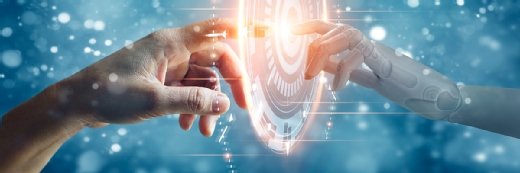
Getty Images
Machine Learning Model May Help Optimize Therapeutic Antibodies
Researchers have created a machine learning tool to help ensure that therapeutic antibodies bind target antigens, repel each other, and ignore non-antigen molecules.
Researchers at the University of Michigan have developed a machine learning (ML) model to help optimize therapeutic antibodies and make them less prone to binding non-target molecules, according to a study published this month in Nature Biomedical Engineering.
Antibody treatments can be used to activate the immune system to fight a variety of diseases, making therapeutic antibodies a key aspect of drug development research. However, these antibodies can bind with molecules that aren’t markers of disease, or even with other antibodies, making them less effective.
The ML tool is designed to help address this by identifying problem areas in an antibody and potential corrections.
“We can use the models to pinpoint the positions in antibodies that are causing trouble and change those positions to correct the problem without causing new ones,” explained corresponding author Peter Tessier, PhD, the Albert M. Mattocks Professor of Pharmaceutical Sciences, Chemical Engineering, and Biomedical Engineering at the University of Michigan, in the press release. “The models are useful because they can be used on existing antibodies, brand new antibodies in development, and even antibodies that haven’t been made yet.”
Antibodies work to combat disease by binding antigens—markers found on the outside of bacteria, viruses, proteins, allergens, parasites, tumor cells, and normal cells—that help identify foreign agents within the body.
Once an antibody binds an antigen, it can signal the body’s immune cells to attack the foreign agent or directly inactive the virus or cells on its own.
Therapeutic antibodies developed and optimized in labs are designed to help fight off serious and hard-to-treat conditions like Alzheimer’s, Parkinson’s, and colorectal cancer, by targeting specific antigens the research team indicated.
However, these antibodies can quickly bind to each other and non-antigen molecules once injected into the body, making them less effective.
“The ideal antibody should do three things at once: bind tightly to what they’re supposed to, repel each other and ignore other things in the body,” Tessier noted.
Antibodies that do not meet these criteria are unlikely to become successful drugs, the press release stated. However, many current clinical-stage antibodies do not meet the criteria, either, demonstrating the need for improved antibody optimization.
To bridge this gap, the researchers evaluated 80 clinical-stage molecules, finding that 75 percent interacted with the wrong molecules, with each other, or both.
Adjusting an antibody’s amino acids and 3D structure could address these issues, but each antibody has hundreds of changeable amino acid positions. Further, these adjustments could cause additional issues when tested in the lab.
“Exploring all the changes for a single antibody takes about two workdays with our models, which is substantially shorter compared to experimentally measuring each modified antibody—which would take months, at best,” said Emily Makowski, the study’s first author and a recent PhD graduate in pharmaceutical sciences at the University of Michigan.
To address these challenges, the research team turned to ML. Each algorithm was trained on experimental data collected on the 80 clinical-stage antibodies to identify how changes in each antibody could help them achieve the three criteria Tessier outlined.
Overall, the model was able to identify these potential changes with 78 to 88 percent accuracy, which the researchers noted could help narrow down the number of necessary antibody changes required for optimization in the lab.
They also noted that moving forward, ML may play a key role in drug development, as biotech companies are already leveraging these tools to optimize therapeutic antibodies and support drug development.
“Some companies have developed antibodies that they are really excited about because they have a desired biological activity, but they know they are going to have problems when they try to use these antibodies as drugs,” said Tessier. “That’s where we come in and show them the specific spots in their antibodies that need to be fixed, and we are already helping out some companies do this.”
Other institutions are also exploring how artificial intelligence (AI) may drive innovations in drug development.
In May, a research team at Ohio State University shared that they had developed a generative AI framework to generate chemical reactions for particular molecules.
The tool, known as G2Retro, is designed to streamline the retrosynthetic analysis, or retrosynthesis, process. Retrosynthesis is a critical step in the drug development pipeline that enables chemists to create potential drugs by identifying a drug target’s molecules and working backward to the chemical reactions that would create the desired molecules.
G2Retro makes this typically laborious process more efficient by using ML to generate reactant structures with the potential to synthesize desired target molecules.