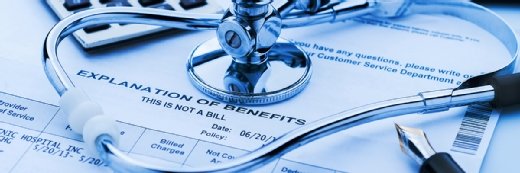
Christian Delbert - stock.adobe.
ML Helps Identify Variations in Individualized Normal Temperature Ranges
A machine learning model determined that age, sex, height, weight, and time of day are factors that contribute to variations in oral temperature measurements.
Machine learning (ML) may help define personalized temperature norms and improve the clinical utility of oral temperature measurements, according to a study published this month in JAMA Internal Medicine.
Oral temperature is often assessed during medical encounters, but the researchers indicated that the “normal” temperature range is poorly defined.
To address this, the research team set out to determine normal oral temperature ranges for individuals in an outpatient setting based on multiple factors—sex, age, time of day, weight, and height—with the help of ML.
The researchers tested this by applying an unsupervised ML algorithm to clinical visit information from 618,306 adult outpatient encounters that took place from April 28, 2008, to June 4, 2017. Data extracted from these encounters included sex, age, time of day, month, height, weight, and primary diagnoses and medications.
The Laboratory Information Mining for Individualized Thresholds (LIMIT) filtering algorithm was used to remove encounters with primary diagnoses that were overrepresented in the tails of the temperature distribution, which left only those diagnoses unrelated to temperature.
The remaining encounters were analyzed using mixed-effects modeling to flag factors associated with normal oral temperature ranges. These factors were then leveraged to generate individualized normal temperature ranges.
Approximately 35.92 percent of the original cohort was removed by LIMIT, as these encounters included diagnoses or medications disproportionately represented in the tails of the temperature distribution. Those encounters removed from the upper tail were associated with infectious disease, while those removed from the lower tail were associated with type 2 diabetes.
After these encounters were removed, 396,195 remained for the final analysis, representing 126,705 patients.
Before applying LIMIT to the original cohort, the average overall oral temperature was 36.71°C. After applying LIMIT, the average temperature fell to 36.64°C. Notably, the average normal oral temperature did not reach 37°C for any subgroup within the study cohort.
The findings also suggested that sex, age, time of day, weight, and height are the factors that contribute significantly to variations in individualized normal temperature ranges.
Overall, using these factors, the researchers found that the average oral temperatures ranged from 36.24°C for the coolest individuals to 36.89°C for the warmest.
These findings led the research team to conclude that normal oral temperature varies in an expected manner based on individual and temporal factors, which may allow individualized normal temperature ranges to be established.
The potential clinical significance of oral temperature values outside of the usual range is an area for future study, they noted.
This research is the latest to suggest that ML and other advanced analytics technologies may be a boon for personalizing care.
In 2019, researchers from Columbia University and Northwell Health demonstrated that deep learning (DL) methods could help develop personalized stress predictors.
Stress contributes to a host of health conditions, and providers are looking for ways to better identify and address stress in an effort to bolster patient wellness.
The research team leveraged a combination of wearable sensor data and qualitative responses from participants to develop the DL model, which was able to predict stress levels with between 75 percent and 85 percent accuracy.