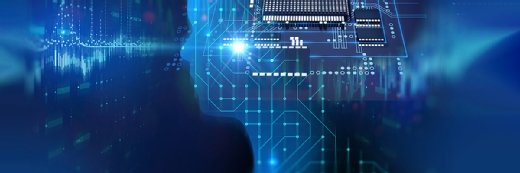
Getty Images
ML Predicts Suicidal Ideation Among Sexual, Gender Minority Individuals
A machine learning model using ecological momentary assessment data could forecast 1-, 3-, and 8-month suicidal ideation in sexual and gender minority individuals.
Researchers demonstrated that a machine learning (ML) model could effectively predict short- and long-term suicidal ideation in sexual and gender minority individuals using ecological momentary assessment data, per a study published recently in JAMA Network Open.
Suicidality is a significant public health concern, especially among those in sexual and gender minority populations. The research team noted that previous studies have evaluated the role of chronic stressors in suicidal ideation, but the impact of daily experiences has not been as well-studied.
To address this, the researchers set out to assess the extent to which ecological momentary assessment information—specifically contextual stressful events and mood fluctuations—can forecast short- and long-term suicidal ideation in these populations.
They leveraged data collected twice a day on stressful events and mood states before, during, and after the Chinese Lunar New Year in 2022. Follow-up surveys evaluating suicidal ideation were then conducted one, three, and eight months later.
The study cohort comprised 103 Chinese individuals aged 18 to 29 years who self-identified as members of sexual and gender minorities and who were not previously diagnosed with psychotic disorders. Those who would find it difficult to participate in the study based on “objective factors,” such as having an irregular sleep rhythm or not having a phone, were also excluded.
Data from these participants were then used to develop ML methods for predicting suicidal ideation. Three models were tested based on which data they were trained on: a baseline approach using chronic stress baseline data; an ecological momentary assessment approach using dynamic patterns of mood states and stressful events; and an ecological momentary assessment plus baseline approach using a combination of baseline data and dynamic patterns.
Of the 103 participants, 19 reported suicidal ideation at baseline. At one, three, and eight months follow-up, 25, 30, and 32 participants reported suicidal ideation, respectively.
The ML models’ performance varied based on the training data and the prediction timeline.
At one-month follow-up, the ecological momentary assessment approach achieved higher performance than the baseline and ecological momentary assessment plus baseline approaches. The approach also showed slightly better performance when predicting suicidal ideation at three and eight months follow-up.
The researchers also found that the prediction effect of feelings faded over time, but the prediction effect of contextual events stayed strong as time went on.
The research team concluded that these findings highlight the potential for detecting suicidal ideation based on analyses of mood and contextual event data. The research also underscores the importance of these risk factors as experienced by sexual and gender minority individuals at different points in time.
The researchers noted that in the future, ML may help identify at-risk individuals and bolster personalized prevention programs to tackle suicidality.
This study is the latest assessing the utility of advanced technologies to combat suicide.
In February, researchers from Worcester Polytechnic Institute (WPI) and Harvard Medical School-affiliated McLean Hospital developed an artificial intelligence (AI) algorithm capable of predicting suicide attempts among study participants and identifying those most at-risk of entering a suicidal mindset.
The AI revealed patterns of broad dissociative symptoms often attributable to trauma and a lack of connection between one’s sense of self and the environment.
The tool could then home in on the dissociative symptoms that best predicted suicide risk.