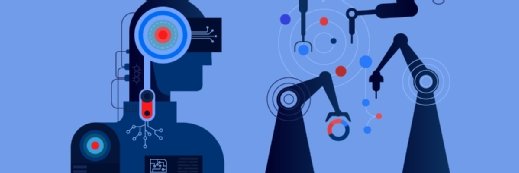
Getty Images
Locally Run Large Language Models May Help Preserve Patient Privacy
Researchers have demonstrated that a locally run large language model may be useful for extracting data from text-based radiology reports while safeguarding privacy.
Researchers from the National Institutes of Health Clinical Center (NIH CC) found that a locally run, privacy-preserving large language model (LLM) may be suitable for labeling radiography reports, according to a study published last week in Radiology.
While LLMs like ChatGPT have recently been lauded for their ability to generate human-like text responses, their healthcare applications are limited by patient data privacy constraints.
“ChatGPT and GPT-4 are proprietary models that require the user to send data to OpenAI sources for processing, which would require de-identifying patient data,” explained senior author Ronald M. Summers, MD, PhD, senior investigator in the Radiology and Imaging Sciences Department at the NIH, in a press release detailing the research. “Removing all patient health information is labor-intensive and infeasible for large sets of reports.”
To address this, the research team assessed a locally run LLM’s ability to label critical findings on chest X-rays.
The model, known as Vicuna-13B, was given 25,596 chest X-ray reports from the NIH and 3,269 reports from a publicly available dataset of de-identified electronic health records (EHRs) called the Medical Information Mart for Intensive Care (MIMIC) Database.
The LLM was tasked with identifying and labeling the presence or absence of 13 key findings: “‘atelectasis,’ ‘cardiomegaly,’ ‘consolidation,’ ‘edema,’ ‘enlarged cardiomediastinum,’ ‘fracture,’ ‘lung lesion,’ ‘lung opacity,’ ‘pleural effusion,’ ‘pleural other,’ ‘pneumonia,’ ‘pneumothorax,’ and ‘support devices.’”
Vicuna’s performance was then compared to those of two existing non-LLM labeling tools, CheXpert and CheXbert.
Overall, the LLM’s performance was comparable to current reference standards, allowing the researchers to achieve moderate to substantial agreement among Vicuna and the currently used labeling tools to which it was compared.
The research team indicated that these findings highlight the potential of LLMs to extract important text-based information from sources like medical records and radiology reports.
“My lab has been focusing on extracting features from diagnostic images,” Summers said. “With tools like Vicuna, we can extract features from the text and combine them with features from images for input into sophisticated AI models that may be able to answer clinical questions.
“LLMs that are free, privacy-preserving, and available for local use are game changers,” he continued. “They're really allowing us to do things that we weren't able to do before.”
Others are also investigating the potential of artificial intelligence (AI) tools in radiology.
In March, a research team assessed the clinical impact of autonomous radiograph reporting using a commercially available AI tool.
The tool is designed to classify each radiograph based on whether it is normal or abnormal. These classifications were then compared to those of radiologists.
In doing so, the team revealed that the interpretation of up to 7.8 percent of radiographs in the dataset could be safely automated using the AI.