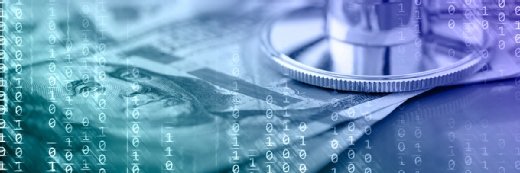
Viorika/istock via Getty Images
Mount Sinai Develops AI Algorithm to Estimate Brain Age Acceleration
The “HistoAge” model can flag areas of the brain vulnerable to age-related changes and predict age at death within 5.45 years by assessing histological images.
Researchers at Mount Sinai have developed an artificial intelligence (AI) tool that can estimate histopathological brain age, predict age at death, and identify areas of the brain vulnerable to age-related changes, according to a study published this month in Acta Neuropathologica.
The research team indicated that as people age, their brains undergo cellular and structural changes that can lead to declines in function and increased susceptibility to neurodegenerative diseases, such as Alzheimer's.
Insights into age acceleration in the brain—the difference between a patient’s chronological and biological age—can be used to explain changes related to disease and functional decline.
To better estimate age acceleration, the researchers developed “HistoAge,” a multiple instance learning algorithm.
The research team gathered nearly 700 digitized slide images of human hippocampal sections from aged brain donors to develop the tool, as the hippocampus plays a role in brain aging and neurodegenerative diseases.
Using these data, the model was trained to estimate each patient's age at death based on these images, a task that the researchers noted would be impossible for a human to do accurately. The discrepancy between the patient’s actual age and the model-predicted age was then used to calculate the amount of age acceleration present.
Compared to existing age acceleration estimation approaches, such as DNA methylation, the age acceleration estimations derived from HistoAge had stronger associations with clinical and pathological outcomes related to cerebrovascular disease, cognitive impairment, and abnormal levels of Alzheimer’s-type degenerative protein aggregation.
The research team concluded that the HistoAge model has significant potential to help clinicians better determine brain age and understand the factors that contribute to neurodegeneration as patients age.
“This model opens the floodgates for a slew of fascinating and essential analyses that bring us closer to finally understanding the aging brain and age-related brain diseases such as Alzheimer's,” said Gabriel A. Marx, MD, MS, resident in Neurology at Icahn Mount Sinai, in the press release. “This is the first time we have been able to put a number to how much aging there is in the brain in pathology. With this approach, we can discover genes that protect against brain aging or genes that make aging worse in the brain, as well as discover the environmental risk factors that make individuals' brains age faster.”
Moving forward, the researchers are working toward developing a multicenter dataset that can be used to train other AI models for brain disease research.
“Using the latest computational approaches, like AI, on human tissue samples from Mount Sinai's vast and diverse collections is a shift in the way we assess human diseases,” explained Kurt W. Farrell, PhD, assistant professor of Pathology, Molecular and Cell-Based Medicine, Neuroscience, and Artificial Intelligence and Human Health at Icahn Mount Sinai. “Our novel HistoAge model is just one example of the way AI is paving the way for further discovery about the mechanisms of aging and neurodegeneration. Clinical scientists are increasingly using AI in research and diagnostic settings. It’s a tool that is revolutionizing medicine and we are excited to be leaders in this space, optimizing machine learning—not to replace our Health System’s commitment to compassionate care, but to improve diagnosis and treatment for all patients.”
This research is one of Mount Sinai’s latest efforts to advance data analytics and AI in healthcare.
Last week, teams from Icahn Mount Sinai and the University of California San Diego (UCSD) were awarded $8.5 million to establish a data integration hub designed to advance National Institutes of Health (NIH) Common Fund initiatives.
These initiatives gather patient data to support biomedical research, including the development of novel therapeutics for a variety of diseases.
To improve the interoperability of these data, the NIH created the Common Fund Data Ecosystem (CFDE). The success of CDFE’s pilot led the agency to award grants to establish two data centers: the CFDE Data Resource Center and the CFDE Knowledge Center.
Mount Sinai and UCSD will lead the CFDE Data Resource Center, which aims to help researchers better utilize the data produced by Common Fund initiatives.