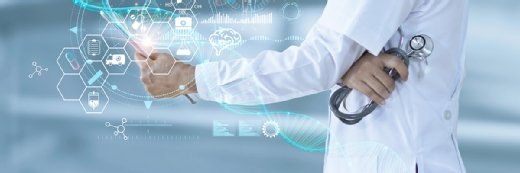
Getty Images/iStockphoto
Cedars-Sinai Deep Learning Tool Accurately Predicts Atrial Fibrillation
New AI model uses routinely acquired outpatient 12-lead electrocardiograms to help clinicians identify abnormal heart rhythms in patients not yet showing symptoms.
Researchers from the Smidt Heart Institute at Cedars-Sinai demonstrated that a deep learning (DL) algorithm can accurately detect signs of atrial fibrillation in patients without symptoms, according to a study published recently in JAMA Cardiology.
Early detection of atrial fibrillation is critical to prevent adverse outcomes like stroke and other cardiovascular complications. The condition is one of the most common types of heart rhythm disorders, the research team indicated, but estimates indicate that one in three people with the condition are unaware that they have it.
In the past, DL tools have been successfully applied to electrocardiograms (ECGs) for the early identification of multiple cardiovascular conditions. However, the researchers noted that much of this research has focused on white patient populations.
To combat this, the research team aimed to develop an algorithm that could detect atrial fibrillation across diverse populations.
To develop such a model, the researchers trained their tool to analyze outpatient 12-lead ECGs. The tool was trained using ECG readings gathered from patients at two Veterans Affairs (VA) health networks from January 1, 1987, to December 31, 2022.
Using these data, the tool was tasked with predicting the presence of atrial fibrillation within 31 days of sinus rhythm ECGs.
The model was then tested on additional ECGs that were held out from the training dataset, along with ECGs from four other VA facilities and Cedars-Sinai.
Overall, the model predicted atrial fibrillation with high accuracy. When tested on the VA data, the tool achieved an area under the receiver operating characteristic curve (AUROC) of 0.86, an accuracy of 0.78, and an F1 score of 0.30.
On the Cedars-Sinai dataset, the model achieved an AUROC of 0.93, an accuracy of 0.87, and an F1 score of 0.46.
These findings led the researchers to conclude that their tool could help identify atrial fibrillation and prevent adverse events across diverse patient groups.
“This study of veterans was geographically and ethnically diverse, indicating that the application of this algorithm could benefit the general population in the U.S.,” said Sumeet Chugh, MD, director of the Division of Artificial Intelligence in Medicine in the Department of Medicine and medical director of the Heart Rhythm Center in the Department of Cardiology, in a press release. “This research exemplifies one of the many ways that investigators in the Smidt Heart Institute and the Division of Artificial Intelligence in Medicine are using AI to address preemptive management of complex and challenging cardiac conditions.”
In the future, the research team plans to continue evaluating the algorithm as part of prospective clinical trials. In doing so, the researchers aim to assess whether the tool can effectively identify patients at high risk for heart attack and stroke.
Other institutions are also investigating how artificial intelligence can help predict cardiovascular event risk.
This week, researchers at Vanderbilt University Medical Center (VUMC) shared how an AI tool deployed at the Monroe Carell Jr. Children’s Hospital can accurately predict blood clot risk in pediatric patients. However, clinicians were often hesitant to follow the model’s treatment recommendations.
Upon investigation, the research team found that clinicians were reluctant to trust the AI because following its recommendation to initiate anti-thrombolytic therapy could lead to bleeding complications.
No bleeding complications were observed among study cohorts, but the researchers noted that the insights from both the accuracy of the algorithm and clinicians’ hesitation to follow its recommendations are crucial to determining whether an AI tool is safe and effective.