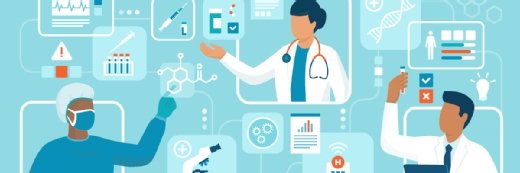
Getty Images
AI Tool May Help Breast Cancer Patients Avoid Unnecessary Chemotherapy
A Northwestern University artificial intelligence tool can combine cancerous and non-cancerous cell patterns to predict the course of invasive breast cancer.
Researchers from Northwestern University have developed an artificial intelligence (AI) tool that may be able to spare breast cancer patients unnecessary chemotherapy treatments by using cell patterns to predict the course of the disease, according to a study published this week in Nature Medicine.
The American Cancer Society estimates that one in eight women in the United States will be diagnosed with breast cancer in her lifetime, and the disease accounts for roughly 30 percent of all new female cancers annually.
Breast cancer is typically diagnosed via diagnostic imaging or biopsy, and once diagnosed, the stage and grade of the cancer are determined. The grading process involves a pathologist assessing the cancer tissue to gauge how abnormal it is, which then informs a patient’s treatment plan.
However, the grading process relies heavily on assessing the appearance of cancer cells, which can be difficult for clinicians. Further, non-cancerous cells have been shown to play a key role in a cancer’s growth, making them useful for prognosis.
Taking these considerations into account, the researchers sought to build an AI model that could evaluate digital images of breast tissue, the appearance of the cancerous and non-cancerous cells present, and how these cells interact.
“These patterns are challenging for a pathologist to evaluate as they can be difficult for the human eye to categorize reliably,” said corresponding study author Lee Cooper, PhD, associate professor of pathology at Northwestern University Feinberg School of Medicine, in a news release. “The AI model measures these patterns and presents information to the pathologist in a way that makes the AI decision-making process clear to the pathologist.”
The tool assesses 26 properties of each patient’s breast tissue in order to output an overall prognostic score. The model is also designed to generate individual cancer, immune, and stromal cells scores to help the pathologist better understand the overall score. These insights could then be used to develop personalized treatment plans for patients.
The research team further noted that the tool has the potential to improve the accuracy of risk assessments for individual breast cancer cases and help evaluate how a patient is responding to treatment. This could allow care teams to adjust the duration or intensity of treatments like chemotherapy where appropriate.
“We also hope that this model could reduce disparities for patients who are diagnosed in community settings,” Cooper stated. “These patients may not have access to a pathologist who specializes in breast cancer, and our AI model could help a generalist pathologist when evaluating breast cancers.”
In the future, the researchers aim to prospectively validate the model for clinical use. The team is also working to create AI models applicable to other invasive breast cancers, such as triple-negative or HER2-positive, as tissue patterns may vary across cancers.
“This will improve our ability to predict outcomes and will provide further insights into the biology of breast cancers,” Cooper explained.
Other AI tools have also been developed recently to predict cancer outcomes.
This month, a research team from the UCLA Health Jonsson Comprehensive Cancer Center detailed how an AI model can successfully predict cancer survival outcomes by assessing epigenetic factors.
The researchers found that by evaluating the gene expression patterns of epigenetic factors in tumors, each factor could then be categorized. This information was then inputted into an AI tool, which used that data to predict outcomes across cancer types.
The model was able to predict cancer outcomes better than some traditional metrics, such as cancer grade and stage.