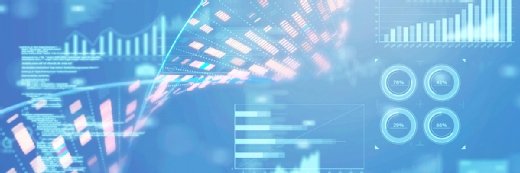
Yuichiro Chino/Moment via Getty
Large Language Model May Help Better Identify Postpartum Hemorrhage
The large language model Flan-T5 was 95 percent accurate in identifying patients with postpartum hemorrhage, which may help predict those most likely to develop it.
Researchers from Brigham and Women’s Hospital have determined that a large language model (LLM) may be more accurate at identifying patients with postpartum hemorrhage than standard methods, according to a recent study published in npj Digital Medicine.
Stanford Medicine estimates that approximately one to five percent of patients experience postpartum hemorrhage. The condition typically occurs shortly after the patient has given birth, but it can occur later as well.
There are multiple potential causes for postpartum hemorrhage and numerous factors that can put some patients at higher risk of developing it, making identifying and tracking the condition a challenge.
To better understand the risk factors for postpartum hemorrhage and the subpopulations impacted by it, the research team turned to artificial intelligence (AI).
“We need better ways to identify the patients that have this complication, as well as the different clinical factors associated with it,” said corresponding author Vesela Kovacheva, MD, of the Department of Anesthesiology, Perioperative and Pain Medicine at Brigham and Women’s Hospital, in the press release discussing the study’s findings. “There are so many amazing large language models being developed right now, and this approach could be used with other conditions and diseases.”
The researchers trained the LLM known as Flan-T5 to recognize concepts associated with postpartum hemorrhage in electronic health record (EHR) data, then tasked the model with flagging patients with the condition.
The LLM was given discharge summaries from 131,284 patients who gave birth at Mass General Brigham hospitals between 1998 and 2015. The model accurately identified patients with postpartum hemorrhage without the need for manual data labeling by the researchers, and the tool outperformed standard methods, which rely on using billing codes to track the condition.
“We looked at all of the patients that Flan-T5 identified as having postpartum hemorrhage and looked at what fraction of those also had the corresponding billing code. It turns out that Flan-T5 was 95 percent accurate and allowed us to identify 47 percent more patients than we would have from the billing codes alone,” said first author Emily Alsentzer, PhD, a research fellow at Brigham and Women’s Hospital. “Ideally, we would like to be able to predict who will develop postpartum’ hemorrhage before they do so, and this is a tool that can help us get there.”
The researchers noted that the approach could be applied to future studies and potentially provide clinical decision support.
Moving forward, the research team plans to apply their methodology to other pregnancy complications in an effort to combat the United States’ maternal health crisis.
This work is part of a larger trend to utilize AI and analytics to improve maternal and infant health.
In a May interview with HealthITAnalytics, Yolande Pengetnze, MD, a pediatrician and vice president of Clinical Leadership at Parkland Center for Clinical Innovation (PCCI) and Parkland Hospital in Dallas, Texas, discussed how the organization is tackling preterm birth disparities through analytics-based risk stratification and patient education.