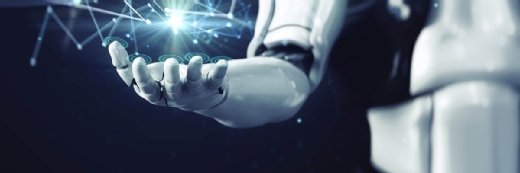
Getty Images
Predictive Model Accurately Flags Postpancreatectomy Hemorrhage Risk
A new risk model can accurately predict late postpancreatectomy hemorrhage in patients with postoperative pancreatic fistula following pancreatoduodenectomy.
Researchers have developed and validated a predictive model capable of identifying late postpancreatectomy hemorrhage (PPH) risk in patients with postoperative pancreatic fistula (cr-POPF) following pancreatoduodenectomy, according to a new study published last week in JAMA Network Open.
The research team indicated that PPH as a result of POPF is a potentially life-threatening complication after undergoing pancreatoduodenectomy. However, there is currently no tool to predict patients at high risk of late PPH.
To address this, the researchers set out to build a risk model to predict PPH using perioperative data. The tool was developed using patient data from 139 participants with clinically relevant POPF who underwent pancreatoduodenectomy between January 1, 2009, and May 20, 2023. The model was then validated using cohort data from a similar group of 154 patients who underwent pancreatoduodenectomy January 1, 2012, to May 31, 2022.
The research team derived a multivariate prediction model — the hemorrhage risk score (HRS) — from analyses of the development cohort’s data. Of 35 variables with known predictive value for PPH, four risk factors were identified and incorporated into the tool: sentinel bleeding, drain fluid culture positive for Candida species, and radiologic proof of rim enhancement of or gas within a peripancreatic fluid collection.
Using these predictors, each patient was assigned to either the low- or high-risk categories for PPH using a point system designed to incorporate the four risk factors. Two points were allocated for sentinel bleeding, while the other factors were each allocated one point. Patients were categorized as low risk if they received one point or fewer, whereas any patient who received two points or more was considered high risk for PPH.
In the development cohort, approximately 30 percent of patients had at least one risk factor, while 11 percent had at least two. The most common risk factor was fluid collection with gas, which impacted 27 percent of the cohort. Conversely, sentinel bleeding was found to be relatively rare, affecting only 14 percent of participants.
Across both the development and validation cohorts, 191 participants were classified as low risk, and 102 were labeled as high risk, but only 74 patients developed late PPH after an average of 13 days.
The model’s ability to discriminate between PPH and no PPH was analyzed using area under the curve (AUC), and the tool demonstrated high performance with an AUC of 0.97. The accuracy of the HRS was also verified using the Brier score, achieving a high prediction value of 0.05. Patients with PPH were predicted accurately in both patient cohorts.
The researchers concluded that these findings demonstrate the tool’s potential to facilitate early identification of patients at high risk for PPH.
Advanced analytics tools are also being leveraged to predict adverse outcome risks in other clinical domains.
Last week, a research team from Brigham and Women’s Hospital demonstrated that a large language model (LLM) known as Flan-T5 may outperform standard methods of identifying patients with postpartum hemorrhage.
Postpartum hemorrhage has a variety of potential causes and associated risk factors, making the potentially life-threatening condition a challenge to identify and track. By gaining insights into the risk factors for postpartum hemorrhage and the subpopulations impacted by it, clinicians may be able to better predict which patients are most likely to be affected.
To facilitate this, the researchers trained Flan-T5 to recognize concepts associated with postpartum hemorrhage in electronic health record (EHR) data and directed it to flag patients with the condition. The LLM was 95 percent accurate, outperforming traditional methods of identifying postpartum hemorrhage patients.