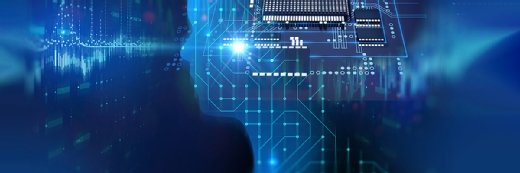
Getty Images
Deep-Learning Model Predicts Physician Burnout Using EHR Logs
Researchers from Washington University in St. Louis have developed a deep-learning model that can predict physician burnout using clinical actions and timestamps from EHR logs.
Researchers have created a deep-learning prediction model that can identify physician burnout by pulling automatically generated EHR activity logs, which may be more convenient than standard approaches for measuring burnout.
Physician burnout is a huge challenge for many health systems, with one 2018 report indicating that 83 percent of clinicians and healthcare leadership see it as a problem in their organizations. In the wake of the COVID-19 pandemic, burnout has grown, and its related costs have increased.
Addressing this issue is a top priority for healthcare organizations, many of whom rely on surveys given to clinicians to evaluate and tackle burnout. However, the researchers point out that many clinicians do not complete these surveys because they are either too busy or are not comfortable revealing that information.
The researchers set out to develop a burnout evaluation method that was as effective as a survey without creating additional burdens for physicians. Previous research has shown that workload was significantly correlated with burnout — the heavier a clinician’s workload, the more likely they were to be experiencing burnout.
Since much of a physician’s workload involves working on EHRs to document and manage clinical activities, the researchers decided to investigate whether EHR activity logs could shed light on burnout.
“These logs can be used to measure workload and tell us how much time one spends on various tasks in an electronic health record, when they perform those tasks, and more,” said Chenyang Lu, PhD, a professor of computer science and engineering who led the study, in a press release. “If we could predict burnout based on this information readily available in the electronic health record, our model could be deployed in most hospitals and predict physician burnout in an unobtrusive and timely fashion.”
EHRs maintain a log of all activity by users, including logins, reading reports, reviewing lab results, and typing notes, in addition to over 2,000 other actions. The press release states that a provider generates 1,000 to 8,000 actions per shift and 90,000 per month. These data points can be used to evaluate provider focus, concentration, and behavior patterns, which can be correlated with burnout.
For their study, the researchers developed a deep-learning framework known as the Hierarchical burnout Prediction based on Activity Logs, or HiPAL. To test HiPAL, they gathered data from the EHR activity logs of 88 resident physicians at Barnes-Jewish Hospital and fellows at the Washington University School of Medicine.
Overall, HiPAL achieved high performance. The research team is optimistic about the framework’s potential applications in healthcare and plans to conduct further research and validation for HiPAL in the future.
This research adds to the growing trend of leveraging AI to combat healthcare worker burnout.
Earlier this month, Maryland-based TidalHealth and AI software company Regard launched a collaboration to reduce provider burnout and enhance patient safety.
In February, researchers from the American Society of Anesthesiologists found that AI-based scheduling improved physician engagement and work-life balance.