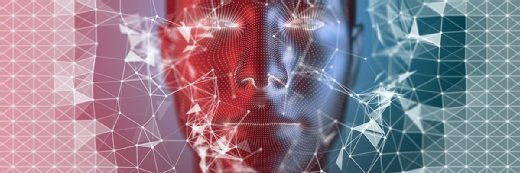
Getty Images
Framework to Address Algorithmic Bias in Healthcare AI Models
An expert panel recently determined that mitigating algorithmic bias requires healthcare stakeholders to promote health equity, transparency, and accountability.
A panel of experts convened by the Agency for Healthcare Research and Quality (AHRQ) and the National Institute on Minority Health and Health Disparities (NIMHD) to address the issue of algorithmic bias in healthcare recently identified five key principles that stakeholders must prioritize across each stage of an algorithm’s life cycle.
Healthcare algorithms, conceptualized in the framework as “mathematical models used to inform decision-making,” are leveraged in multiple use cases, such as diagnosis, treatment, triage, risk stratification, and resource allocation.
However, the presence of bias in these tools can perpetuate care disparities, creating a major hurdle to their implementation. A growing body of research has determined that many clinical algorithms contain biases of some kind – such as racial bias in diabetes prediction tools and gaps in data sources – that can inadvertently, but negatively, impact health equity efforts.
The researchers underscored that biases in healthcare algorithms are present across specialties, often to the detriment of marginalized racial and ethnic groups, in addition to other disadvantaged groups like people with lower incomes.
“Many health care algorithms are data-driven, but if the data aren’t representative of the full population, it can create biases against those who are less represented,” explained Lucila Ohno-Machado, MD, PhD, MBA, the Waldemar von Zedtwitz Professor of Medicine and deputy dean for biomedical informatics at Yale School of Medicine, who co-chaired the panel, in a press release. “As the use of new AI techniques grows and grows, it will be important to watch out for these biases to make sure we do no harm to specific groups while advancing health for others. We need to develop strategies for AI to advance health for all.”
To prevent and mitigate these biases, the panel developed a framework for designed to center health equity across the five steps of a healthcare algorithm’s life cycle: problem formulation; data selection, assessment, and management; algorithm development, training, and validation; deployment and integration of algorithms in intended settings; and algorithm monitoring, maintenance, updating, or de-implementation.
The framework is split into five guiding principles for stakeholders looking to utilize healthcare algorithms.
The first directs healthcare organizations to promote health equity at all phases of the algorithm life cycle, while the second is designed to ensure that algorithms and their use are both explainable and transparent.
The third principle advises stakeholders to engage patients and communities during all phases of the model’s life cycle to build trust. The fourth guideline encourages leadership to explicitly identify fairness issues and trade-offs that come along with an algorithm’s use, and the fifth directs users to establish accountability for fairness and equity in the outcomes of the algorithm.
With these principles, the authors posited that healthcare stakeholders can partner to create systems, processes, and standards to prevent and address algorithmic bias.
“Algorithmic bias is neither inevitable nor merely a mechanical or technical issue. Conscious decisions by algorithm developers, algorithm users, health care industry leaders, and regulators can mitigate and prevent bias and proactively advance health equity,” they wrote.