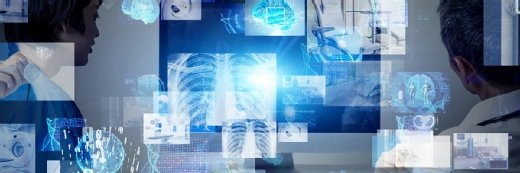
metamorworks/istock via Getty Im
Precision Pathology Artificial Intelligence May Improve Cancer Treatment
A new artificial intelligence tool designed to interpret gene activity within medical images may provide insights into the pathology of diseases like cancer.
Researchers from the Perelman School of Medicine at the University of Pennsylvania have developed an artificial intelligence (AI)-based medical imaging technique capable of analyzing the gene activity found within tissue microenvironments, which may have significant impacts on precision medicine and pathology, according to a study published last week in Nature Biotechnology.
The tool, known as Inferring Super-Resolution Tissue Architecture (iStar), is designed to provide both detailed views of individual cells and a broader understanding of how a patient’s genes operate. The research team believes that the tool could help clinicians better diagnose and treat cancers that could go undetected using traditional methods.
Using the tool, clinicians can observe cancer cells that may have been virtually invisible when relying on current imaging and pathology methodologies. iStar is capable of automatically detecting tertiary lymphoid structures (TLS), tissues that drive immune responses in a host of chronic diseases, including cancer.
The presence of these structures correlates with how well a cancer patient is likely to respond to immunotherapy and their likelihood of cancer survival. However, identifying the presence of TLS can be a challenge, as it requires high levels of precision.
The researchers posited that iStar could help to determine which patients are most likely to benefit from immunotherapy. The tool integrates insights from spatial transcriptomics – an emerging field focused on mapping gene activities within the tissue microenvironment – with histology.
The tool uses machine learning (ML) to “break down” medical images to find clinically relevant fine details, then broadens to analyze larger tissue patterns. Then, the tool utilizes this information to predict gene activity at the individual cell level.
“The power of iStar stems from its advanced techniques, which mirror, in reverse, how a pathologist would study a tissue sample,” explained Mingyao Li, PhD, a professor of Biostatistics and Digital Pathology who led the research, in a press release. “Just as a pathologist identifies broader regions and then zooms in on detailed cellular structures, iStar can capture the overarching tissue structures and also focus on the minutiae in a tissue image.”
To evaluate the tool, the researchers applied it to a combination of breast, kidney, colorectal, and kidney cancer images, mixed with images of healthy tissues. The tool was able to accurately flag tumor and cancer cells that were difficult to identify with the naked eye.
iStar also demonstrated that it could perform these tasks significantly faster than similar tools. When tested on one of the breast cancer datasets, the tool completed its analysis in nine minutes, while its highest-performing competitor took over 32 hours to do the same.
The researchers hope that iStar may be able to provide support to clinicians in detecting and diagnosing more hard-to-identify cancers.
“The implication is that iStar can be applied to a large number of samples, which is critical in large-scale biomedical studies,” Li said. “Its speed is also important for its current extensions in 3D and biobank sample prediction. In the 3D context, a tissue block may involve hundreds to thousands of serially cut tissue slices. The speed of iStar makes it possible to reconstruct this huge amount of spatial data within a short period of time.”
Moving forward, the research team will apply the tool to biobanks with much larger image sample databases to determine if iStar could help researchers better understand tissue microenvironments and develop enhanced diagnosis and treatment protocols.
The research reflects a growing interest in AI-aided medical imaging technologies.
Last week, a research team from Case Western Reserve University was awarded a four-year, $1.1 million grant from the National Science Foundation (NSF) to design enhanced medical imaging AI.
The project focuses on making medical imaging safer and more efficient by developing an AI-based alternative that does not rely on the use of chemical “contrast agents” to improve diagnostic accuracy. Traditional imaging processes, such as computed tomography (CT), magnetic resonance imaging (MRI), and X-rays leverage these agents, making them cost- and resource-intensive.
To help health systems maintain care quality, the researchers will design an AI tool that utilizes only non-contrast images.