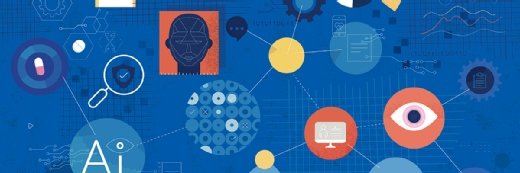
DrAfter123/DigitalVision Vectors
Deep Learning Model Accurately Detects Cardiac Function, Disease
A deep learning model can classify left ventricular ejection fraction, aortic stenosis, tricuspid regurgitation, and other conditions from chest radiographs.
A deep learning (DL) model has been developed and validated to estimate cardiac function and valvular disease using chest radiographs, according to a study published this month in The Lancet Digital Health.
The study indicates that while chest radiographs provide a common and widely available option for cardiovascular examination and certain cardiac structures are visible using this method, the ability of chest radiographs to help classify valvular disease and cardiac function is not well understood.
This led researchers to develop and validate a DL model capable of simultaneously detecting left ventricular ejection fraction, tricuspid regurgitant velocity, mitral regurgitation, aortic stenosis, aortic regurgitation, mitral stenosis, tricuspid regurgitation, pulmonary regurgitation, and inferior vena cava dilation using chest radiographs.
To do so, the research team gathered chest radiographs and associated echocardiogram data from four medical institutions in Japan. Data from three of these were used for training, validation, and internal testing of the model. The fourth site’s data was used for external testing.
The training data included 22,551 chest radiographs associated with 22,551 echocardiograms. These were obtained from 16,946 patients. The external testing data included 3,311 chest radiographs from 2,617 patients.
From there, the DL model’s performance was evaluated in terms of its area under the receiver operating characteristic curve (AUC), sensitivity, specificity, and accuracy.
The AUCs, accuracy, sensitivity, and specificity for the model were 0.92, 86 percent, 82 percent, and 86 percent for classifying the left ventricular ejection fraction; 0.85, 75 percent, 83 percent, and 73 percent for classifying the tricuspid regurgitant velocity; 0.89, 85 percent, 82 percent, and 85 percent for classifying mitral regurgitation; 0.83, 73 percent, 79 percent, and 72 percent for classifying aortic stenosis; 0.83, 68 percent, 88 percent, and 67 percent for classifying aortic regurgitation; 0.86, 90 percent, 83 percent, and 90 percent for classifying mitral stenosis; 0.92, 83 percent, 87 percent, and 83 percent for classifying tricuspid regurgitation; 0.86, 69 percent, 91 percent, and 68 percent for classifying pulmonary regurgitation; and 0.85, 86 percent, 73 percent, and 87 percent for classifying inferior vena cava dilation.
These findings led the researchers to conclude that the DL model is capable of accurately estimating valvular heart diseases and cardiac functions using digital chest radiographs. Additionally, they indicated that the model can classify echocardiography values in a fraction of the time while relying on low system requirements, suggesting that it has potential for use in areas with a shortage or absence of echocardiography specialists.
This research reflects a growing interest in leveraging artificial intelligence-based tools to improve cardiovascular care.
In June, researchers from the University of Pittsburgh Medical Center (UPMC) announced the development of a machine learning (ML) solution designed to leverage electrocardiogram (ECG) readings to detect and classify heart attacks.
The model helps address the problem of unclear ECGs in chest pain triage, which can delay critical medical care.
The tool detects subtle patterns in the ECG that clinicians may miss and classifies patients based on their heart attack risk. The research team found that the model accurately reclassified one in three chest pain patients using this methodology.