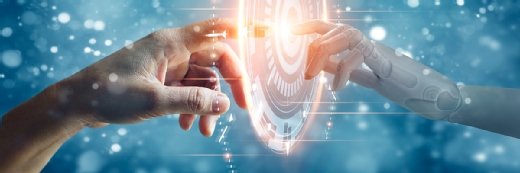
Getty Images
$4M Grant to Fund Development of Sleep Apnea Outcome Prediction Tools
Mount Sinai researchers will use a $4 million NIH grant to investigate how artificial intelligence could advance outcome prediction for patients with sleep apnea.
Researchers from Mount Sinai have been awarded $4.1 million from the National Heart, Lung, and Blood Institute (NHLBI) at the National Institutes of Health (NIH) to develop artificial intelligence (AI) models to predict adverse outcomes in obstructive sleep apnea.
The condition, which is caused by improper breathing and intermittent airflow blockage during sleep, is estimated to impact nearly 39 million adults in the United States. Sleep apnea can significantly impair sleep and contribute to adverse outcomes like hypertension, diabetes, long COVID, and all-cause mortality.
Obstructive sleep apnea is diagnosed using the Apnea-Hypopnea Index (AHI), a tool designed to count the number of apneas – when a patient stops breathing during sleep – and hypopneas – periods of reduced airflow during sleep. The number of apneas and hypopneas are then combined to quantify the severity of an individual’s sleep apnea.
However, the AHI is limited and is not necessarily an accurate predictor of patient outcomes, leading clinicians to call for the development of improved diagnostic and prognostic tools.
To that end, the research team at Mount Sinai set out to develop an AI-driven approach to assess obstructive sleep apnea patients’ risk of adverse outcomes. The approach looks at the underlying physiology of the condition and some of the sleep functions it impairs – sleep stages, breathing, and oxygen levels – to generate a risk score that helps predict patients’ short- and long-term outcomes, such as excessive daytime sleepiness, neurocognitive impairment, and cardio-cerebrovascular morbidity.
The researchers developed two machine learning (ML) models to predict patients’ risk by pulling in data on ventilatory, hypoxic, and arousal variables. Following training, the models were used to determine adverse outcome risks in 11,000 sleep apnea patients.
The findings of this analysis revealed that one of the models could accurately predict the probability of sleepiness due to apnea with an accuracy of about 87 percent, whereas a model using the AHI could predict the same metric with an accuracy of only 54 percent.
In a cohort of 4,700 participants, the AI approach could predict cardiovascular mortality with over 80 percent accuracy compared to the standard approach, which achieved only 58 percent accuracy.
“Our proposal uses a state-of-the-art artificial intelligence model that risk-profiles sleep apnea patients using data from routine sleep studies,” said principal investigator Ankit Parekh, PhD, director of the Sleep and Circadian Analysis (SCAN) Group and assistant professor of Medicine (Pulmonary, Critical Care and Sleep Medicine) at the Icahn School of Medicine at Mount Sinai, in a news release.
“Our study will assess the real-world performance of an AI approach and offer crucial evidence needed to translate metrics that go beyond the apnea-hypopnea [index] in assessing severity of obstructive sleep apnea into clinical practice. Achieving this would leave us poised to shift the paradigm in clinical management of obstructive sleep apnea,” Parekh continued.
Moving forward, the research team aims to further evaluate the models in a cohort of Mount Sinai Integrative Sleep Center patients. Participants will undergo polysomnogram sleep studies to record data on their brain waves, oxygen levels, heart rates, and breathing during sleep. The patients will then be monitored and asked to maintain a digital sleep diary for three months following their sleep studies.
The findings of this study will then be retrospectively validated for further statistical analysis.
This is the latest research to explore how digital health tools can improve outcomes for patients with sleep apnea.
Last year, a research team from the Georgia Institute of Technology (Georgia Tech) shared that they developed a wearable device that can detect the condition with 88.5 percent accuracy.
The device, which relies on forehead- and chin-mounted patches, is designed to provide a potential alternative to polysomnography tests, which can be costly and inaccessible to patients.
The wearable sensors transmit signals recording brain, eye, and muscle activity via Bluetooth, and these data are then used to generate a sleep score. The device’s high accuracy indicates that it may have utility for helping detect sleep apnea if clinically validated.