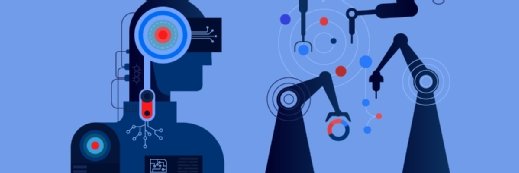
Getty Images
Deep Learning Model Accurately Detects, Predicts Alzheimer's Disease
Novel deep learning model leverages metabolic biomarkers to predict the likelihood that Alzheimer's disease will develop long before clinical symptom onset.
A research team from West Virginia University (WVU) has developed a deep learning model capable of detecting and predicting Alzheimer's disease using metabolic biomarkers.
The researchers sought to determine which biomarkers are most relevant to the condition and use that information to build an artificial intelligence (AI)-based tool to predict whether or not a patient is likely to develop Alzheimer's.
A deep learning approach allows the research team to process and analyze vast volumes of complex data more efficiently than other computational methods.
“The deep learning method using artificial neural networks, which are inspired by the layered structure of the brain’s neurons and their computations, has reached unprecedented prediction performance for complex tasks,” said study lead Kesheng Wang, PhD, professor in the WVU School of Nursing, in the news release. “It has been shown deep learning techniques are more accurate for Alzheimer’s disease diagnosis in comparison to conventional machine learning models.”
The researchers further noted that metabolites can potentially inform disease progression in Alzheimer's, making identifying diagnostic biomarkers a priority.
“Alzheimer’s disease may start years or even decades before clinical symptom onset, therefore it is crucial to identify predictive biomarkers in the preclinical stage so medical science can develop strategies to prevent the progression of the disease,” Wang explained.
Early detection of Alzheimer’s is also key for the development of drug and therapeutic development.
To identify relevant biomarkers, the research team performed feature selection via the Least Absolute Shrinkage and Selection Operator (LASSO) algorithm. This analysis identified 150 potential biomarkers using Ultra Performance Liquid Chromatography Mass Spectrometry (UPLC-MS/MS) data. Of these, 21 were selected as most relevant to Alzheimer’s.
Multiple biomarkers were found to impact hippocampal atrophy, an early sign of Alzheimer's disease and other dementias.
“The metabolites are part of the glucose, amino acid and lipid metabolisms,” Wang said. “Some of the metabolites are correlated with clinical biomarkers — such as plaques — cognitive measures and hippocampus volume associated with Alzheimer’s patients.”
To test the predictive value of these biomarkers, the research team incorporated them into multiple deep learning models and tested the performance of each. The tools were evaluated using information from a cohort of 177 patients in the Alzheimer's Disease Neuroimaging Initiative (ADNI) dataset. Of these individuals, 78 were diagnosed with Alzheimer’s, while the remaining 99 were cognitively normal.
These data were split into 70 percent for training and 30 percent for validation. The highest-performing model achieved an accuracy of 0.881, an F1-score of 0.892, and an area under the curve (AUC) of 0.873.
Despite the success of their model, the researchers emphasized that deep learning approaches for detecting Alzheimer's are still relatively new and that more research is needed.
To that end, the research team plans to continue its work by integrating metabolomic and protein data into other deep learning tools.
“The metabolic basis of Alzheimer’s disease is still poorly understood and the relationships between systemic abnormalities in metabolism and Alzheimer’s disease pathogenesis are unclear,” Wang indicated. “This study shows there is potential to identify metabolic biomarkers that are predictive of Alzheimer’s disease diagnosis and progression.”
Others are also investigating how advanced analytics approaches could help clinicians better conceptualize Alzheimer’s risk and progression.
This month, a research team from the University of Texas at Arlington (UTA) detailed the development of a novel learning-based framework to predict how a patient’s Alzheimer's will progress over time.
The tool is designed to help patients and caregivers anticipate the additional care needs that arise alongside the disease’s progression by pinpointing where an individual is within the Alzheimer's disease-development spectrum.
The framework codes five stages of the condition’s development – normal cognition, significant memory concern (SMC), early mild cognitive impairment (EMCI), late mild cognitive impairment (LMCI), and Alzheimer’s disease – into a “disease-embedding tree” (DETree).
This information can then be used to project a patient’s data onto the tree, which represents their clinical status and the trajectory of their Alzheimer’s progression.