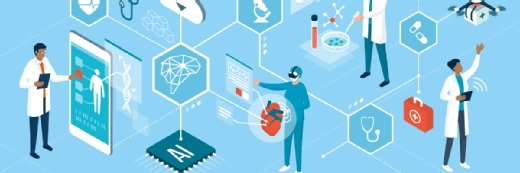
Getty Images
Machine Learning Methods May Improve Brain Tumor Characterization
Researchers combining mass spectrometry with machine learning to assess meningioma tumors found that the tools were 87% accurate in classifying tumor grade.
Researchers from University of Florida (UF) Health have demonstrated that a combination of machine learning (ML) and liquid chromatography-high resolution mass spectrometry (LC-HRMS) can help make brain tumor evaluations more efficient.
The research was published in the Journal of the American Society for Mass Spectrometry, detailing how these tools can refine the metabolomic and lipidomic characterization of meningioma tumors. While these are a common type of brain tumor, accurately assessing them is critical to prevent adverse outcomes.
Meningioma tumors are classified into three categories: grade I, grade II, and grade III. Grade I tumors are typically slow-growing and less threatening, so treatment focuses on tumor removal and follow-up monitoring for the patient. Grade III tumors are more aggressive, requiring both removal and radiation treatment.
Grade II tumors present a challenge for clinicians.
“Grade II tumors are the gray zone,” said study co-author Jesse L. Kresak, MD, a clinical associate professor in the UF College of Medicine’s department of pathology, immunology and laboratory medicine, in the press release. “Do we take [the tumor] out and watch to see if it comes back? Or do we also irradiate the area with the idea of preventing a recurrence?”
This dilemma led the researchers to pursue an approach that improves meningioma tumor evaluation and better guides clinicians’ treatment decisions.
To achieve this, the research team analyzed 85 meningioma samples, obtaining chemical profiles of each tumor’s small molecules and fats. Doing so allowed the researchers to more precisely characterize differences between grades of tumors and identify potential biomarkers that would be helpful for diagnosis.
Initially, the research team had not planned to incorporate ML into their study, instead opting to analyze the byproducts of metabolism within the tumor cells, which would have yielded a chemical “fingerprint” that would help differentiate between benign and malignant tumors.
However, the researchers realized that the incorporation of ML could help provide additional insights.
“After talking about it, we knew that machine learning could be a good opportunity to find things that we wouldn’t be able to find ourselves,” explained Timothy J. Garrett, PhD, a co-author of the paper, an associate professor in the College of Medicine’s department of pathology, immunology and laboratory medicine and a UF Health Cancer Center member.
By utilizing ML, the tumor evaluation process became significantly more efficient. Kresak noted that when she is diagnosing a meningioma tumor, she can assess approximately 20 data points in ten minutes. With ML, 17,000 data points were analyzed in less than a second.
Incorporating ML did not lead to significant dips in accuracy. Of those tested, one of the ML models classified the grades of tumors with 87 percent initial accuracy, which the researchers indicated could be improved with the addition and analysis of more samples.
The research team noted that their findings may be useful for meningioma diagnosis and treatment, as tumors can be reclassified after initial pathologist assessment based on new information about the sample’s genetic makeup.
“We are further understanding different tumors by using these tools. It’s a way to help us get the right treatment for our patients,” Kresak said.
The research is just one example of how health systems are investigating the use of data analytics and artificial intelligence (AI) to bolster oncology.
This month, the University of Texas MD Anderson Cancer Center established its Institute for Data Science in Oncology (IDSO), designed to transform cancer care through the application of clinical expertise and data science.
IDSO is set to focus on collaboration among stakeholders in medicine, science, academia, and industry in an effort to tackle cancer patients’ most urgent needs.
The institute will support enhanced data generation, collection, and management at MD Anderson, leading to advances in personalized care and patient experience.