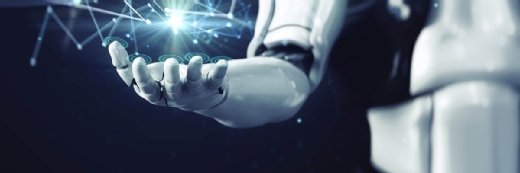
Getty Images
Machine learning predicts perioperative peripheral artery disease risk
Machine learning models accurately forecasted one-year major adverse outcomes following endovascular intervention for peripheral artery disease.
Machine learning (ML) algorithms can accurately predict one-year major adverse limb event (MALE) or death following endovascular intervention for peripheral artery disease (PAD), according to a study published recently in JAMA Network Open.
The research team indicated that endovascular interventions for PAD can carry significant perioperative risks, but current outcome prediction tools are limited.
To address this, the researchers turned to ML.
Data from 235,677 patients who underwent endovascular intervention for PAD between January 1, 2004, and July 5, 2023, with 1 year of follow-up, were pulled from the Vascular Quality Initiative (VQI) for analysis.
Patients treated for trauma, acute limb ischemia, lower extremity aneurysmal disease, dissection or malignant neoplasm, and those with unreported procedure type or symptom status, or undergoing concurrent surgical bypass, were excluded from the study.
The data then were split into a training set containing 70 percent of participants and a test set with the remaining 30 percent of the cohort.
These data were used to identify relevant predictive features: 75 preoperative, 24 intraoperative and 13 postoperative features, for a total of 112.
Preoperative features included preprocedural characteristics – demographics, previous procedures, comorbidities, functional status, medications, anatomy and others – while intraoperative features consisted of procedural characteristics and postoperative factors were related to patients’ in-hospital course and complications.
The research team then used the identified preoperative features and 10-fold cross-validation to train six ML models to forecast one-year MALE – characterized as composite of thrombectomy or thrombolysis, surgical reintervention or major amputation – or death.
Each algorithm’s performance was assessed in terms of area under the receiver operating characteristic curve (AUROC). Following selection of the best-performing preoperative model, the researchers built additional algorithms using intraoperative and postoperative data.
Of the original patient cohort, 71,683 participants developed one-year MALE or death.
The best preoperative prediction model was extreme gradient boosting (XGBoost), achieving an AUROC of 0.94, an accuracy of 0.86, a sensitivity of 0.87, a specificity of 0.85, a positive predictive value of 0.85 and a negative predictive value of 0.87.
The XGBoost model also maintained high performance using intraoperative and postoperative data, with AUROCs of 0.94 and 0.98, respectively.
These results suggest that ML tools can accurately forecast one-year outcomes following endovascular intervention for PAD, indicating that they may have the potential to guide perioperative risk mitigation strategies and improve patient outcomes.
This research comes as stakeholders continue to debate the role of artificial intelligence (AI) and ML in cardiovascular care.
In February, the American Heart Association (AHA) published a statement detailing the current state of AI use in the diagnosis and treatment of cardiovascular disease.
The report outlined potential applications, challenges, limitations of these technologies and how AI may be deployed safely and effectively.
The statement underscored that these tools have significant potential for use cases like medical imaging, but that multiple hurdles – like legal and ethical concerns, lack of protocols for appropriate information sourcing and sharing, the absence of robust regulatory pathways and the need to grow the scientific knowledge base around these technologies – limit their adoption.