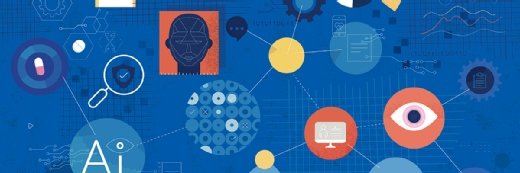
DrAfter123/DigitalVision Vectors
Deep learning model detects COVID-19 infection using lung imaging
A deep neural network-based automated detection tool could assist emergency room clinicians in diagnosing COVID-19 effectively using lung ultrasound images.
Johns Hopkins researchers have developed a deep learning-based model to detect COVID-19 infection using lung ultrasound images, according to a study published recently in Communications Medicine.
The automated detection tool uses deep neural networks (DNNs) to identify COVID-19 features in lung ultrasound B-mode images and may help clinicians diagnose emergency department patients more efficiently.
"We developed this automated detection tool to help doctors in emergency settings with high caseloads of patients who need to be diagnosed quickly and accurately, such as in the earlier stages of the pandemic," said senior author Muyinatu Bell, PhD, an associate professor in the Department of Electrical and Computer Engineering in the Whiting School of Engineering at Johns Hopkins University, in a news release. "Potentially, we want to have wireless devices that patients can use at home to monitor progression of COVID-19, too."
To develop the tool, the research team trained the DNNs on multiple datasets with a variety of images: 40,000 simulated images, 174 publicly available in vivo images, 958 additional in vivo images curated by the researchers and a combination of datasets.
Using these data, the models were tasked with flagging B-lines – bright, vertical image abnormalities that indicate inflammation in patients with pulmonary complications – to diagnose COVID-19 infection. The resulting model achieved high performance in identifying abnormalities associated with COVID-19.
The tool’s success in accurately identifying COVID-19 in lung ultrasound images may indicate that its diagnostic potential could extend to other conditions, like heart failure.
"What we are doing here with AI tools is the next big frontier for point of care," stated co-author Tiffany Fong, MD, an assistant professor of emergency medicine at Johns Hopkins Medicine. "An ideal use case would be wearable ultrasound patches that monitor fluid buildup and let patients know when they need a medication adjustment or when they need to see a doctor."
The tool’s ability to use computer-generated images alongside real ultrasounds is key to its diagnostic capability.
"We had to model the physics of ultrasound and acoustic wave propagation well enough in order to get believable simulated images," Bell explained. "Then we had to take it a step further to train our computer models to use these simulated data to reliably interpret real scans from patients with affected lungs."
When the COVID-19 pandemic began, researchers lacked the real-world data necessary to train artificial intelligence (AI) to diagnose patients, but having a model that can use simulated data may ease burdens associated with a lack of data, the research team noted.
"Early in the pandemic, we didn't have enough ultrasound images of COVID-19 patients to develop and test our algorithms, and as a result our deep neural networks never reached peak performance," said first author Lingyi Zhao, who developed the software while a postdoctoral fellow in Bell's lab. "Now, we are proving that with computer-generated datasets we still can achieve a high degree of accuracy in evaluating and detecting these COVID-19 features."
Research like this underscores the growing role of AI in medical imaging analytics, but the deployment of these technologies is still fraught.
Experts from Harvard Medical School (HMS), the Massachusetts Institute of Technology (MIT) and Stanford University recently found that the use of AI-based tools to assist radiologists can unpredictably impact clinician performance.
The researchers emphasized that there is some evidence to suggest that AI can enhance radiologists’ performance as a whole, but studies looking at the effect of AI use on individual performance are limited.
To bridge the research gap, the team assessed a group of radiologists based on their ability to correctly identify clinically relevant imaging abnormalities with and without the use of AI. The analysis revealed that the impact of the AI was inconsistent, improving performance for some radiologists while worsening it for others.